Any-Shot GIN: Generalizing Implicit Networks for Reconstructing Novel Classes
Yongqin Xian1, Julian Chibane2,3, Bharat Lal Bhatnagar2,3, Bernt Schiele2, Zeynep Akata2,3,4, Gerard Pons-Moll2,31ETH Zurich, Switzerland
2Max Planck Institute for Informatics, Saarland Informatics Campus, Germany
3University of Tübingen, Germany
4Max Planck Institute for Intelligent Systems, Germany
International Conference on 3D Vision 2022 (3DV), 2022 - Oral - Best Paper Honourable Mention
Abstract
We address the task of estimating the 3D shapes of novel shape classes from a single RGB image. Prior works are either limited to reconstructing known training classes or are unable to reconstruct high-quality shapes. To solve those issues, we propose Generalizing Implicit Networks (GIN) which decomposes 3D reconstruction into 1.) front-back depth estimation followed by differentiable depth voxelization, and 2.) implicit shape completion with 3D features. The key insight is that the depth estimation network learns local class-agnostic shape priors, allowing us to generalize to novel classes, while our implicit shape completion network is able to predict accurate shapes with rich details by learning implicit surfaces in 3D voxel space. We conduct extensive experiments on a large-scale benchmark using 55 classes of ShapeNet and real images of Pix3D. We qualitatively and quantitatively show that the proposed GIN significantly outperforms the state of the art on both seen and novel shape classes for single-image 3D reconstruction. We also illustrate that our GIN can be further improved by using only few-shot depth supervision from novel classes.Method Overview
Video
Results on Novel Classes
RGB Input | ONet | SDFNet | Our |
---|---|---|---|
![]() |
|||
![]() |
|||
![]() |
|||
![]() |
|||
![]() |
|||
![]() |
Citation
@inproceedings{Xian2022gin, title = {Any-Shot GIN: Generalizing Implicit Networks for Reconstructing Novel Classes}, author = {Xiang, Yongqin and Chibane, Julian and Bhatnagar, Bharat Lal and Schiele, Bernt and Akata, Zeynep and Pons-Moll, Gerard}, booktitle = {2022 International Conference on 3D Vision (3DV)}, organization = {IEEE}, year = {2022}, }
Acknowledgments
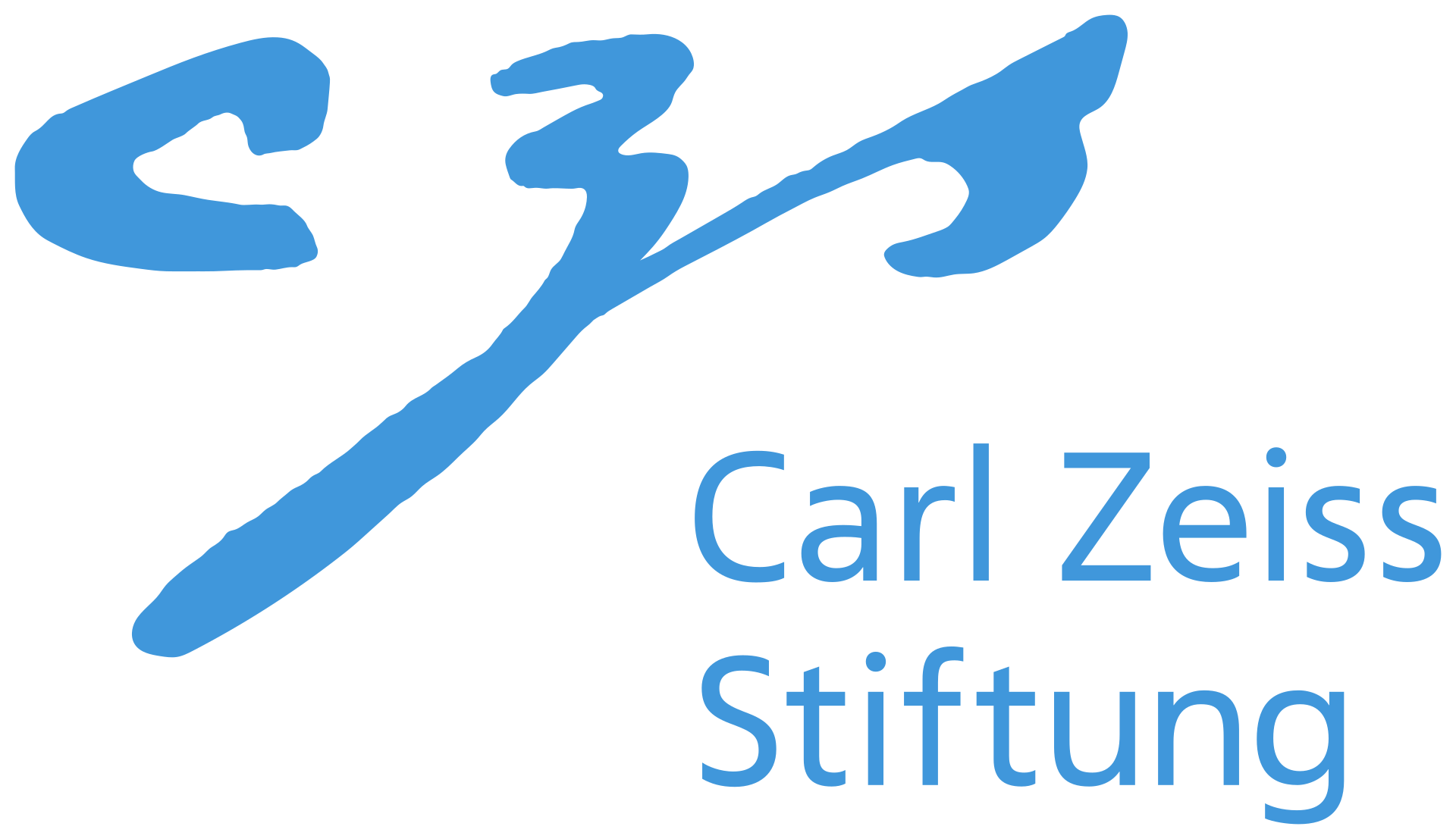
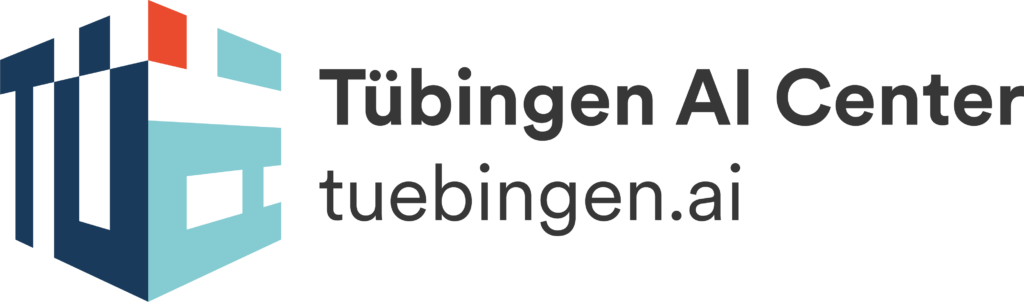
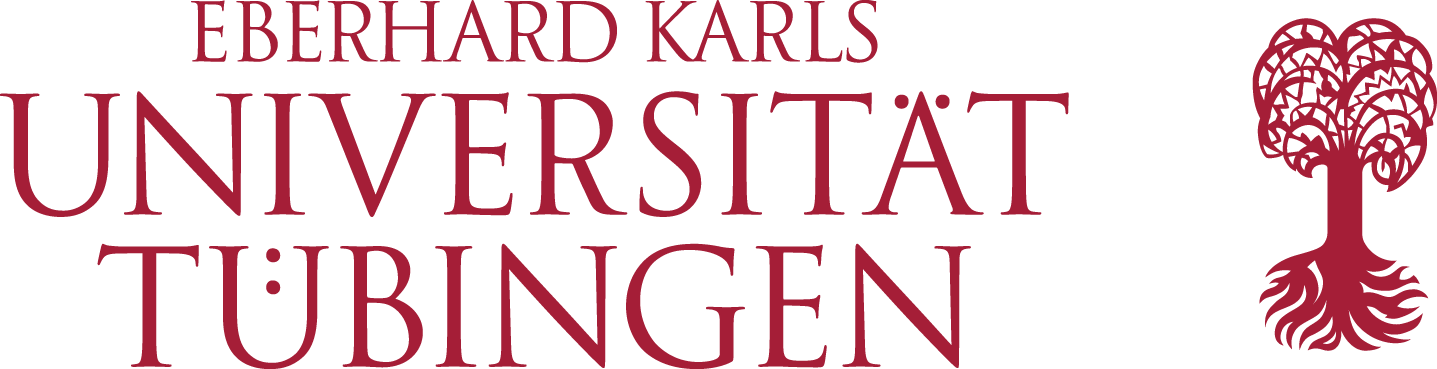
This work is funded by the Deutsche Forschungsgemeinschaft (DFG, German Research Foundation) - 409792180 (Emmy Noether Programme, project: Real Virtual Humans) and the German Federal Ministry of Education and Research (BMBF): Tubingen AI Center, ¨ FKZ: 01IS18039A. G. Pons-Moll is a member of the Machine Learning Cluster of Excellence, EXC number 2064/1, Project number 390727645. J. Chibane is a fellow of the Meta Research PhD Fellowship Program - area: AR/VR Human Understanding The project was made possible by funding from the Carl Zeiss Foundation.