SCENIC: Scene-aware Semantic Navigation with Instruction-guided Control
Xiaohan Zhang, Sebastian Starke , Vladimir Guzov , Zhensong Zhang , Eduardo Pérez Pellitero , Gerard Pons-Moll1University of Tübingen and Tübingen AI Center, Germany 2Max Planck Institute for Informatics, Saarland Informatics Campus, Germany 3Meta Reality Labs Research 4Huawei Noah's Ark Lab
Overview
SCENIC is a text-conditioned scene interaction model. It adapts to complex scenes with varying terrains and also supports user-specified semantic control with natural language. Given a 3D scene, our model takes as cues of user-specified trajectory as sub-goals, and text.

Method
To learn such a model, the key is to use a hierarchical reasoning of the scene. The goal-centric canonicalization reasons about the high-level goal and the altitude difference, and the human-centric distance field reasons about the granular level details.

Besides a hierarchical reasoning of the scene, our diffusion model also leverages frame-wise alignment between motion and the text for seamless transition between motion styles.
Dataset
We train our model on paired motion-scene-text data. By fitting real human motion segments onto synthetic terrains patches, it alleviates the data scarcity by multiplying the effect of data. We select the best-fitted terrains based on contact and penetration.
Generalization and Text-editing
Results are tested on four real-world scene datasets of Replica, Matterport, HPS and Laserhuman. With the goal-centric canonicalization, our model can avoid undesirable penetration and alleviates the floating artifact. Our model can generalize to different scenes and text control prompts. It can even generate "hopping over the stool and sit".
Qualitative Evaluation
It can also seamlessly transit between diverse motion styles controlled by user-specified texts.
Citation
@article{zhang2024scenic, title = {SCENIC: Scene-aware Semantic Navigation with Instruction-guided Control}, author = {Zhang, Xiaohan and Starke, Sebastian and Guzov, Vladimir and Dhamo, Helisa and Pérez Pellitero, Eduardo and Pons-Moll, Gerard}, booktitle = {Arxiv}, year = {2024}, }
Acknowledgments
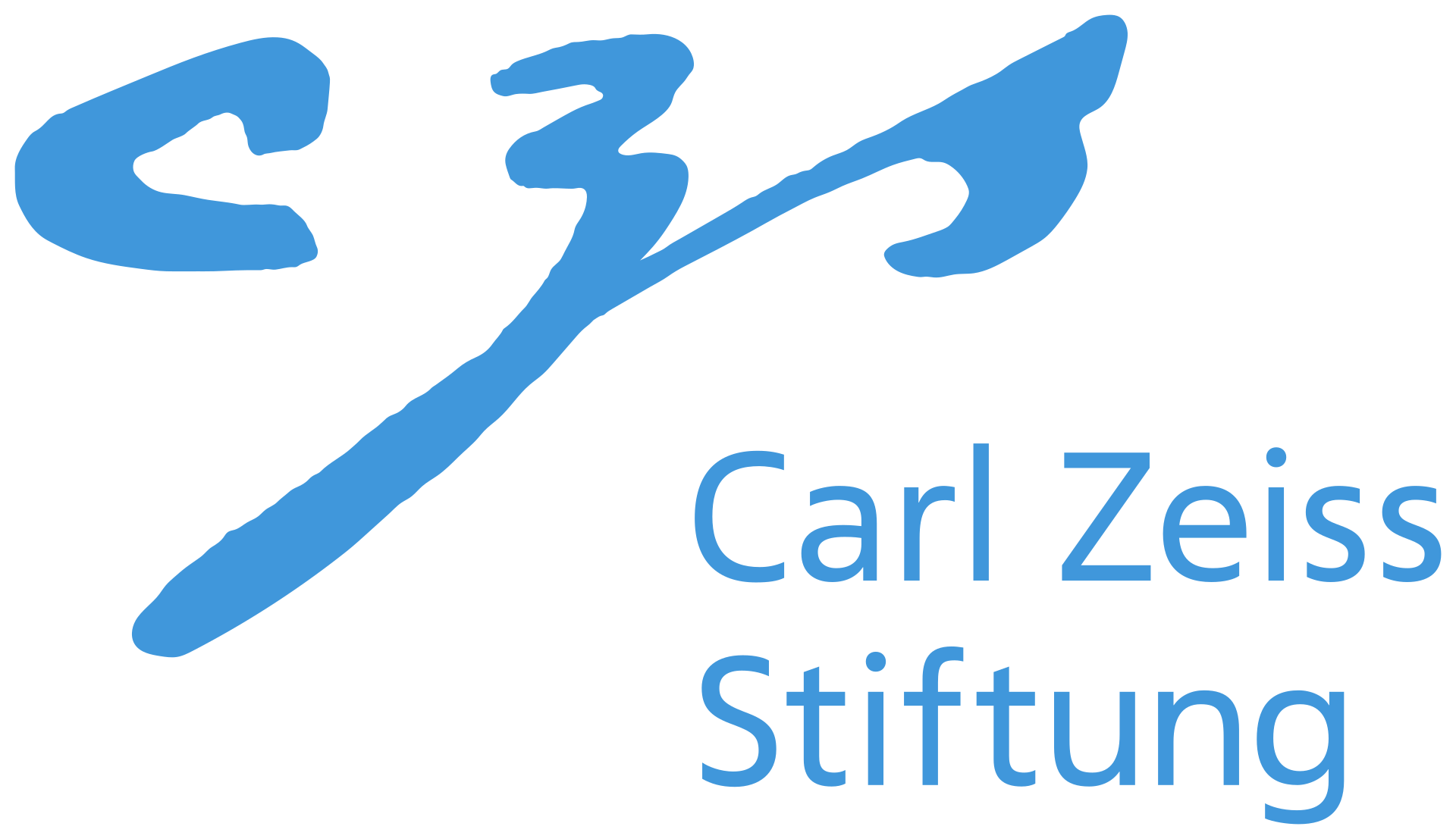
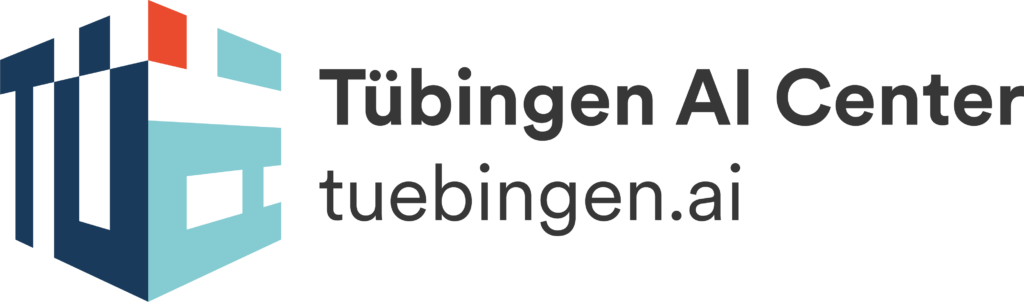
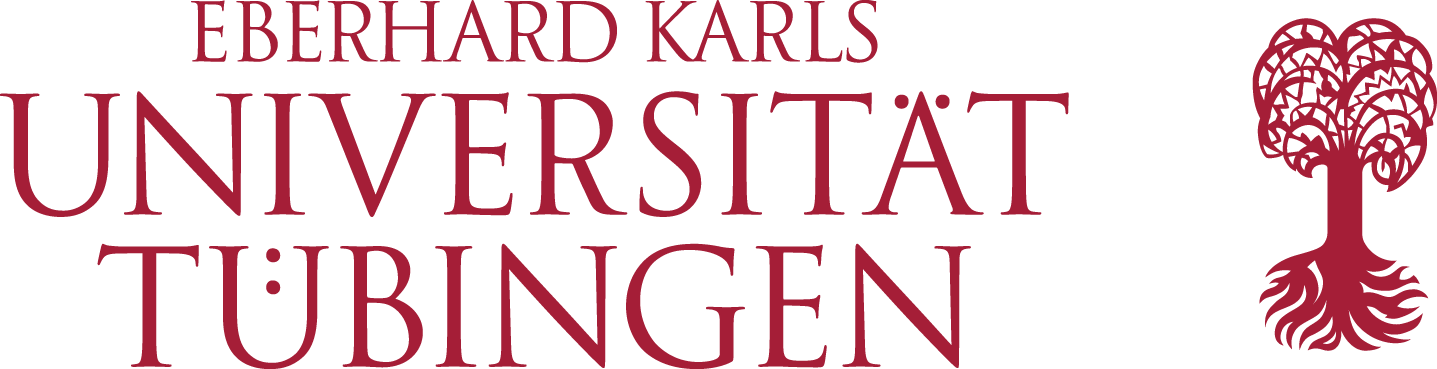
A big thank you goes to Hongwei Yi for the useful discussions and exchanging ideas. We appreciate the RVH group members for their useful feedback. This work is funded by the Deutsche Forschungsgemeinschaft (DFG, German Research Foundation) - 409792180 (Emmy Noether Programme, project: Real Virtual Humans), Huawei, and German Federal Ministry of Education and Research (BMBF): T¨ubingen AI Center, FKZ: 01IS18039A. Gerard Pons-Moll is a member of the Machine Learning Cluster of Excellence, EXC number 2064/1 – Project number 390727645. The project was made possible by funding from the Carl Zeiss Foundation.