FORCE: Dataset and Method for Intuitive Physics Guided Human-object Interaction
Xiaohan Zhang, Bharat Lal Bhatnagar, , Sebastian Starke , Ilya Petrov , Vladimir Guzov , Helisa Dhamo , Eduardo Pérez Pellitero , Gerard Pons-Moll1University of Tübingen and Tübingen AI Center, Germany 2Max Planck Institute for Informatics, Saarland Informatics Campus, Germany 3Meta Reality Labs Research 4Huawei Noah's Ark Lab
Overview

FORCE - a method and dataset for synthesizing diverse, nuanced human-object interactions by modeling the physical attributes of the interaction.

FORCE Dataset
Previous work on synthesizing dynamics object interaction do not consider the physical attributes such as object weight or friction. To study this problem we need a dataset capturing interactions with varying physical attributes. The dataset captures the daily interactions of pushing, pulling and carrying 8 real objects. It features diverse, different styled interactions with 4-6 levels of resistance. We release the over 500 sequences of RGB-D, human and object motion (.npz), and object scans.
Key Insight

We propose a novel intuitive physics encoding. It ensures the model is aware of the interrelation between the applied human force and the different perceived resistances during the interaction. The encoding informs our model the direction in 3D that the force is applied. It also crucially ensures our method is aware of the interplay between the human force and the resistance.
Generalization
Different Shapes
Different Hand Contact Modes
Full Video
Citation
@article{zhang2024force, title = {FORCE: Dataset and Method for Intuitive Physics Guided Human-object Interaction}, author = {Zhang, Xiaohan and Bhatnagar, Bharat Lal and Starke, Sebastian and Petrov, Ilya and Guzov, Vladimir and Dhamo, Helisa and Pérez Pellitero, Eduardo and Pons-Moll, Gerard}, booktitle = {Arxiv}, year = {2024}, }
Acknowledgments
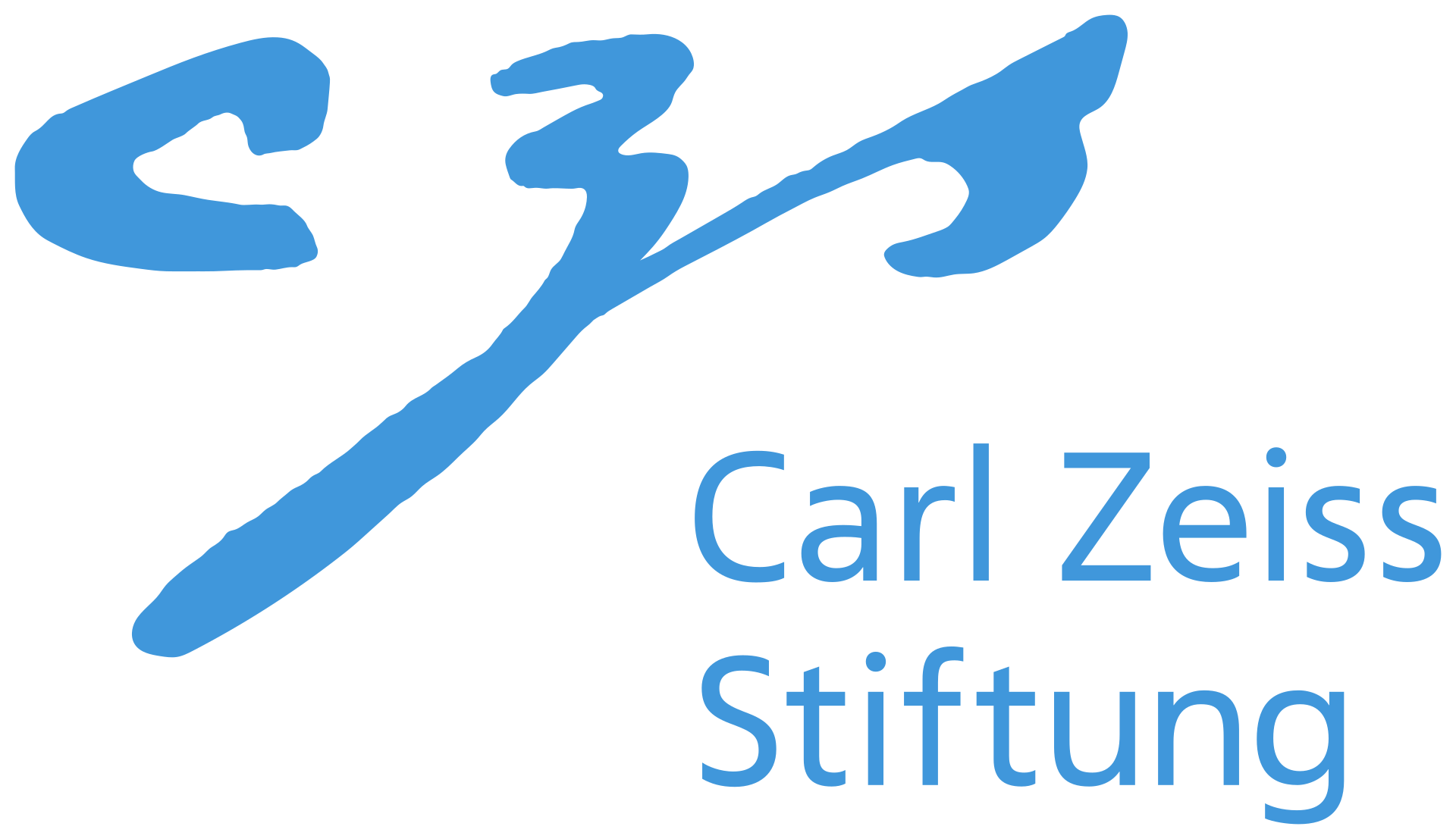
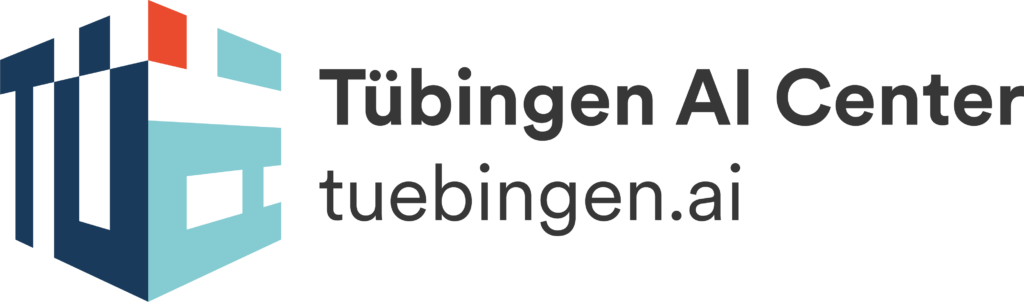
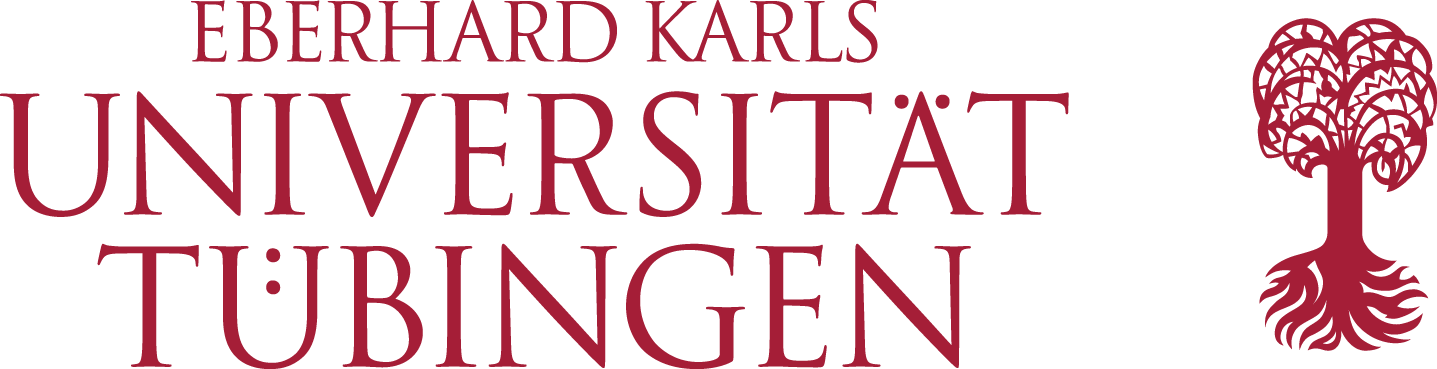
This work is funded by the Deutsche Forschungsgemeinschaft (DFG, German Research Foundation) - 409792180 (Emmy Noether Programme, project: Real Virtual Humans), Huawei, and German Federal Ministry of Education and Research (BMBF): T¨ubingen AI Center, FKZ: 01IS18039A. Gerard Pons-Moll is a member of the Machine Learning Cluster of Excellence, EXC number 2064/1 – Project number 390727645. The project was made possible by funding from the Carl Zeiss Foundation.