BEHAVE: Dataset and Method for Tracking Human Object Interactions
BEHAVE dataset and pre-trained models
Bharat Lal Bhatnagar1,2, Xianghui Xie2, Ilya Petrov1, Cristian Sminchisescu3, Christian Theobalt2and Gerard Pons-Moll1,2 1University of Tübingen, Germany 2Max Planck Institute for Informatics, Saarland Informatics Campus, Germany 3Google Research CVPR 2022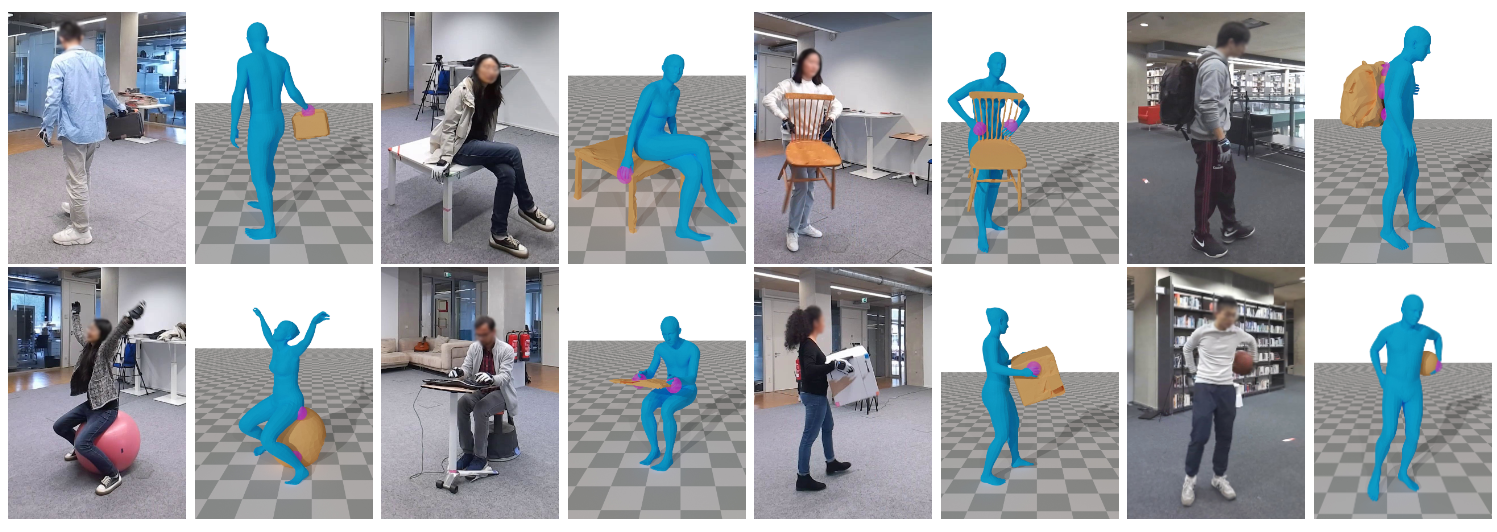
We present BEHAVE dataset, the first full body human-object interaction dataset with multi-view RGBD frames and corresponding 3D SMPL and object fits along with the annotated contacts between them. We use this data to learn a model that can jointly track humans and objects in natural environments with an easy-to-use portable multi-camera setup.
Video
Description
The BEHAVE* dataset is the largest dataset of
human-object interactions in natural environments, with 3D
human, object and contact annotation, to date.
The dataset includes:
- 8 subjects interacting with 20 objects at 5 natural environments.
- In total 321 video sequences recorded with 4 Kinect RGB-D cameras.
- Each frame contains human and object masks and segmented point clouds.
- Every image is paired with 3D SMPL and object mesh registration in camera coordinate.
- Camera poses for every sequence.
- Textured scan reconstructions for the 20 objects.
* formerly known as the HOI3D dataset.
Download
For further information about the BEHAVE dataset and for download links, please click hereUpdates
Citation
If you use this dataset, you agree to cite the corresponding CVPR'22 paper:@inproceedings{bhatnagar22behave, title = {BEHAVE: Dataset and Method for Tracking Human Object Interactions}, author={Bhatnagar, Bharat Lal and Xie, Xianghui and Petrov, Ilya and Sminchisescu, Cristian and Theobalt, Christian and Pons-Moll, Gerard}, booktitle = {{IEEE} Conference on Computer Vision and Pattern Recognition (CVPR)}, month = {jun}, organization = {{IEEE}}, year = {2022}, }
Acknowledgments
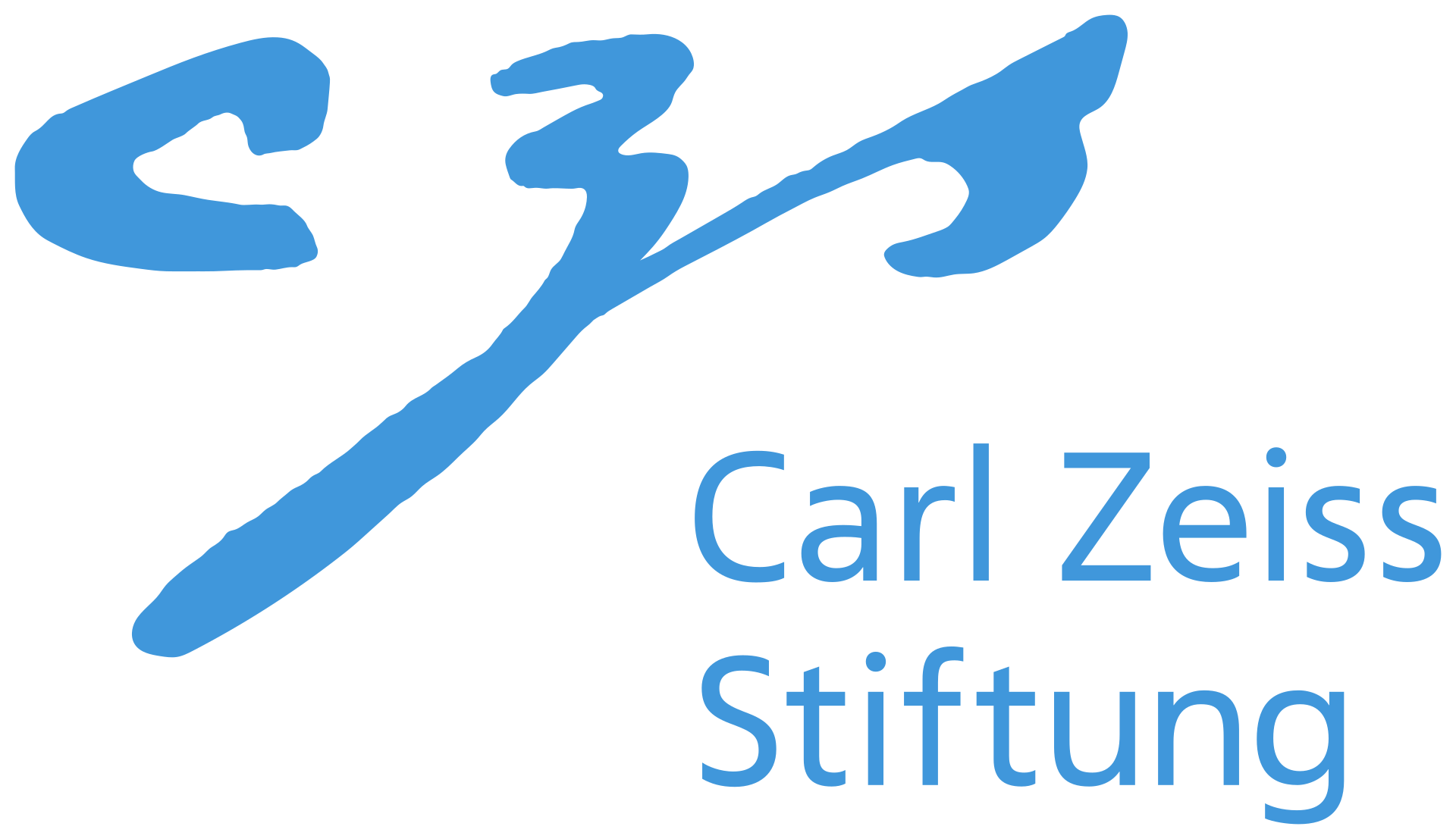
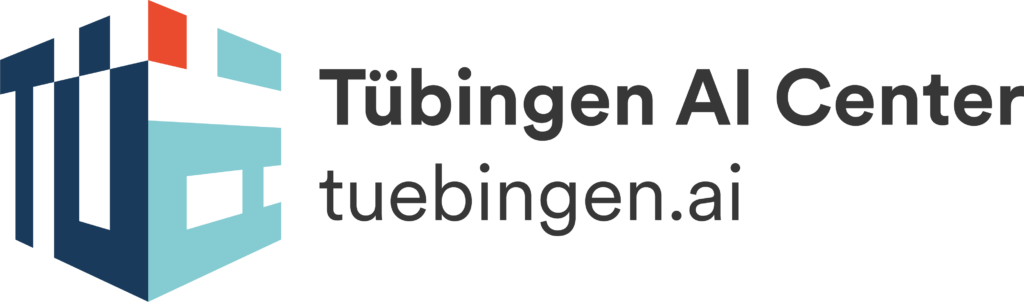
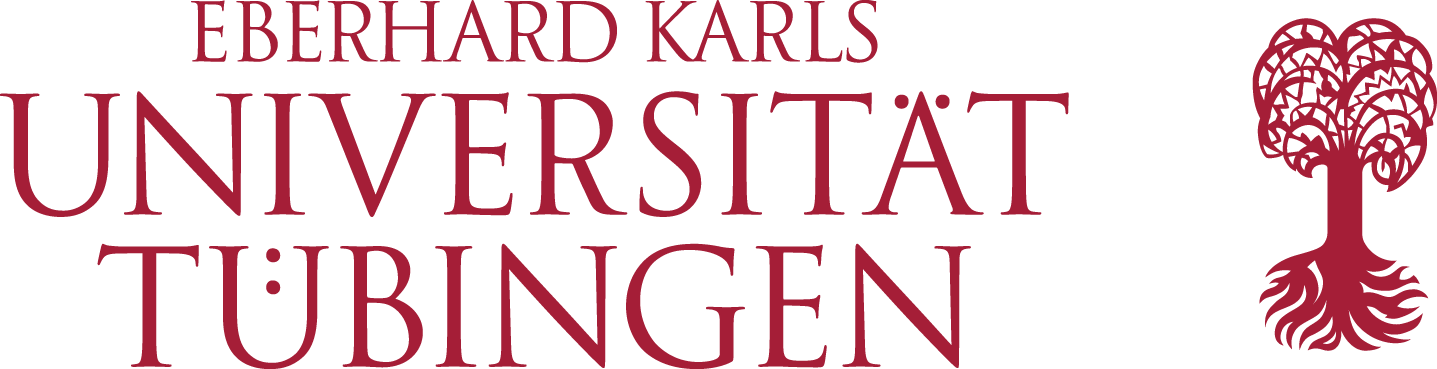
Special thanks to RVH team members, and reviewers, their feedback helped improve the manuscript. This work is funded by the Deutsche Forschungsgemeinschaft (DFG, German Research Foundation) - 409792180 (Emmy Noether Programme, project: Real Virtual Humans), German Federal Ministry of Education and Research (BMBF): Tubingen AI ¨ Center, FKZ: 01IS18039A and ERC Consolidator Grant 4DRepLy (770784). Gerard Pons-Moll is a member of the Machine Learning Cluster of Excellence, EXC number 2064/1 – Project number 390727645. The project was made possible by funding from the Carl Zeiss Foundation.