Adjoint Rigid Transform Network: Task-conditioned Alignment of 3D Shapes
Keyang Zhou 1, 2 , Bharat Lal Bhatnagar 1, 2 , Bernt Schiele 2 , Gerard Pons-Moll 1, 21University of Tübingen, Germany
2Max Planck Institute for Informatics, Saarland Informatics Campus, Germany

Most learning methods for 3D data (point clouds, meshes) suffer significant performance drops when the data is not carefully aligned to a canonical orientation. Aligning real world 3D data collected from different sources is non-trivial and requires manual intervention. In this paper, we propose the Adjoint Rigid Transform (ART) Network, a neural module which can be integrated with a variety of 3D networks to significantly boost their performance. ART learns to rotate input shapes to a learned canonical orientation, which is crucial for a lot of tasks such as shape reconstruction, interpolation, non-rigid registration, and latent disentanglement. ART achieves this with self-supervision and a rotation equivariance constraint on predicted rotations. The remarkable result is that with only self-supervision, ART facilitates learning a unique canonical orientation for both rigid and nonrigid shapes, which leads to a notable boost in performance of aforementioned tasks.
Shape Alignment

Human Registration

A: Input point cloud. B: 3D-CODED (single initialization) C: 3D-CODED (+ART) D: Groundtruth
Shape Interpolation
Video
Citation
@inproceedings{zhou2022art, title = {Adjoint Rigid Transform Network: Task-conditioned Alignment of 3D Shapes}, author = {Zhou, Keyang and Bhatnagar, Bharat Lal and Schiele, Bernt and Pons-Moll, Gerard}, booktitle = {2022 International Conference on 3D Vision (3DV)}, organization = {IEEE}, year = {2022}, }
Acknowledgments
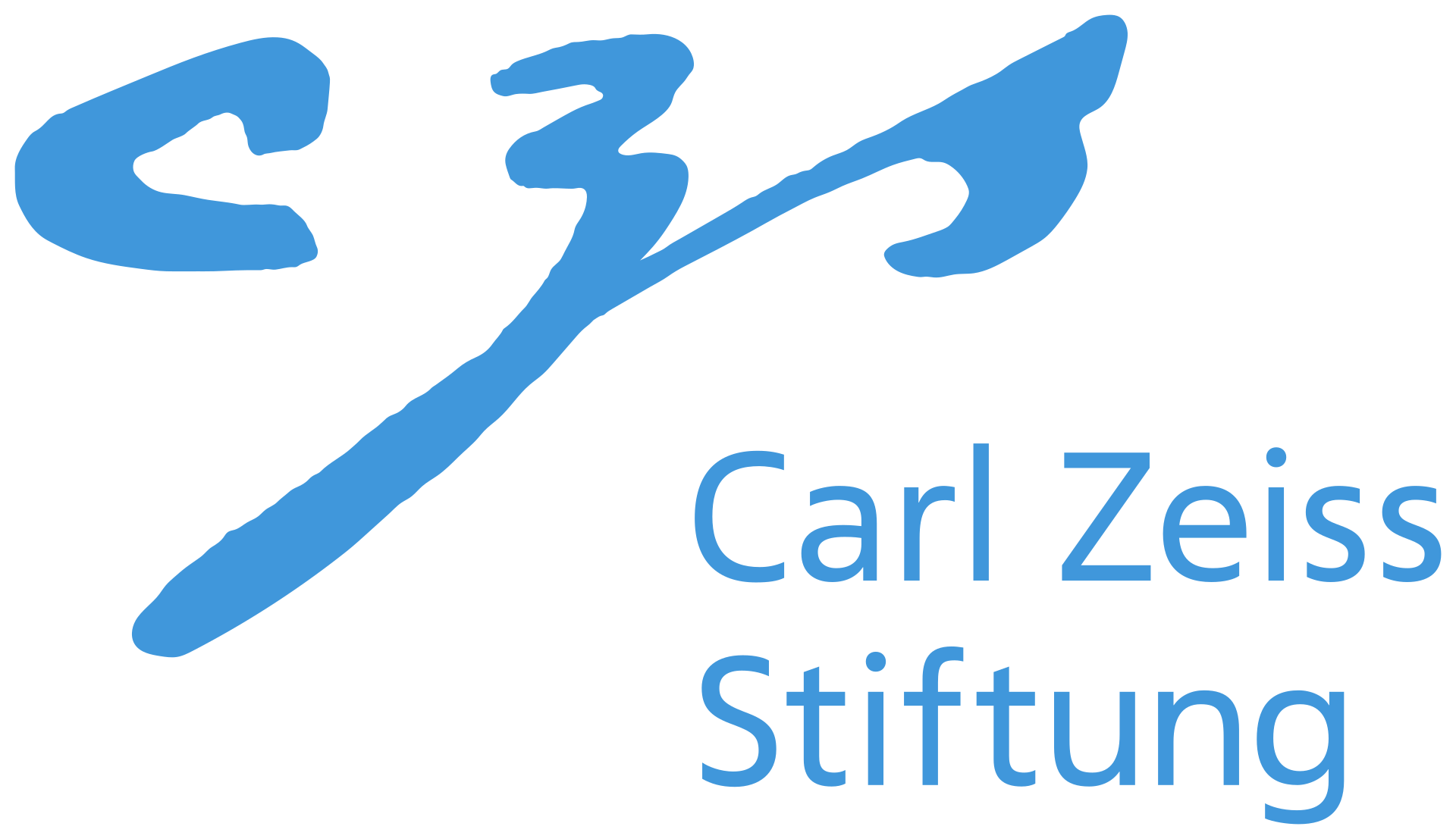
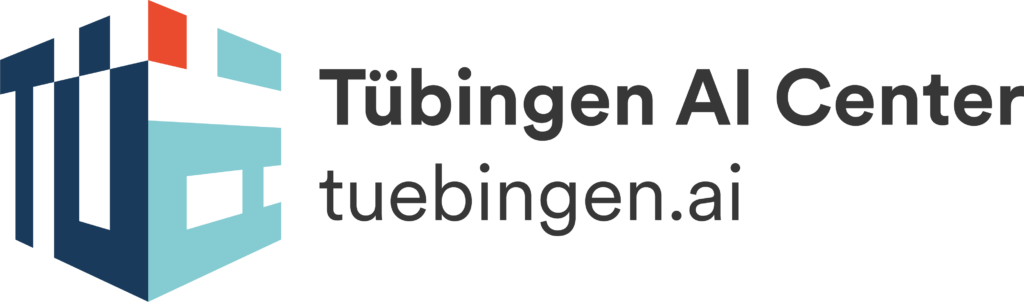
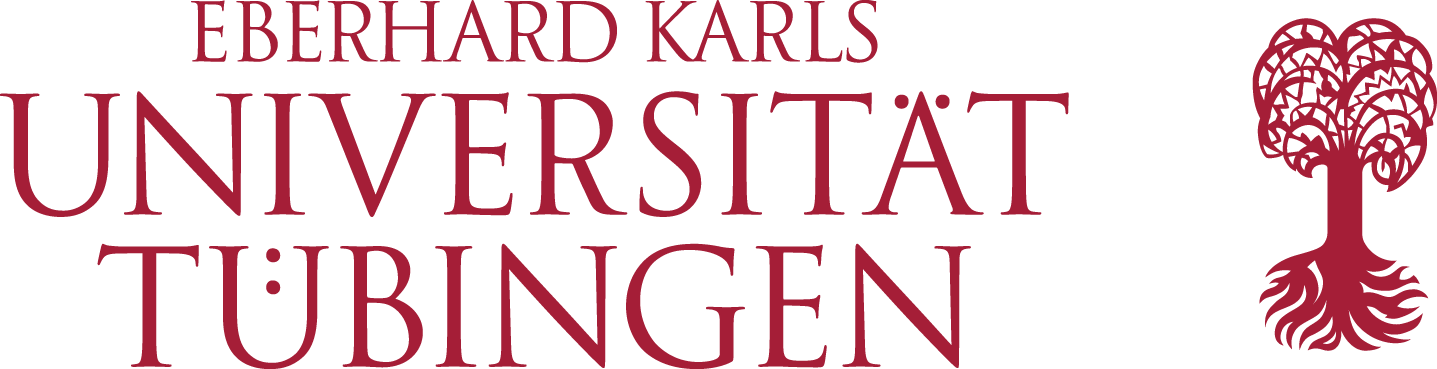
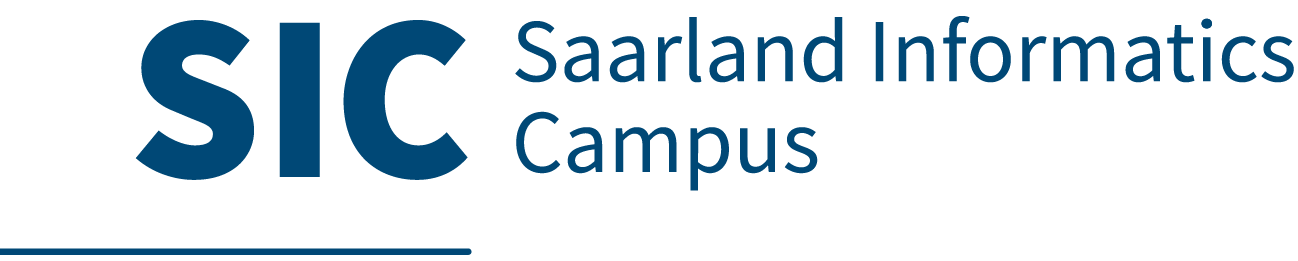
This work is supported by the German Federal Ministry of Education and Research (BMBF): T¨ubingen AI Center, FKZ: 01IS18039A. This work is funded by the Deutsche Forschungsgemeinschaft (DFG, German Research Foundation) - 409792180 (Emmy Noether Programme, project: Real Virtual Humans). Gerard Pons-Moll is a member of the Machine Learning Cluster of Excellence, EXC number 2064/1 – Project number 390727645. The project was made possible by funding from the Carl Zeiss Foundation.