Visibility Aware Human-Object Interaction Tracking from Single RGB Camera
Xianghui Xie1, 2, Bharat Lal Bhatnagar1,2, Gerard Pons-Moll1,21 University of Tübingen, Tübingen AI Center, Germany
2 Max Planck Institute for Informatics, Saarland Informatics Campus, Germany
CVPR 2023, Vancouver
We present VisTracker, an approach to jointly track the human, the object and the contacts between them, in 3D, from a monocular RGB video. Our method is able to track the object and contacts even under heavy occlusions.
Abstract
Capturing the interactions between humans and their environment in 3D is important for many applications in robotics, graphics, and vision. Recent works to reconstruct the 3D human and object from a single RGB image do not have consistent relative translation across frames because they assume a fixed depth. Moreover, their performance drops significantly when the object is occluded. In this work, we propose a novel method to track the 3D human, object, contacts, and relative translation across frames from a single RGB camera, while being robust to heavy occlusions. Our method is built on two key insights. First, we condition our neural field reconstructions for human and object on per-frame SMPL model estimates obtained by pre-fitting SMPL to a video sequence. This improves neural reconstruction accuracy and produces coherent relative translation across frames. Second, human and object motion from visible frames provides valuable information to infer the occluded object. We propose a novel transformer-based neural network that explicitly uses object visibility and human motion to leverage neighboring frames to make predictions for the occluded frames. Building on these insights, our method is able to track both human and object robustly even under occlusions. Experiments on two datasets show that our method significantly improves over the state-of-the-art methods.
Key idea
Long narrated video
Citation
@inproceedings{xie2023vistracker, title = {Visibility Aware Human-Object Interaction Tracking from Single RGB Camera}, author = {Xie, Xianghui and Bhatnagar, Bharat Lal and Pons-Moll, Gerard}, booktitle={IEEE Conference on Computer Vision and Pattern Recognition (CVPR)}, month={June}, year={2023} }
Acknowledgments
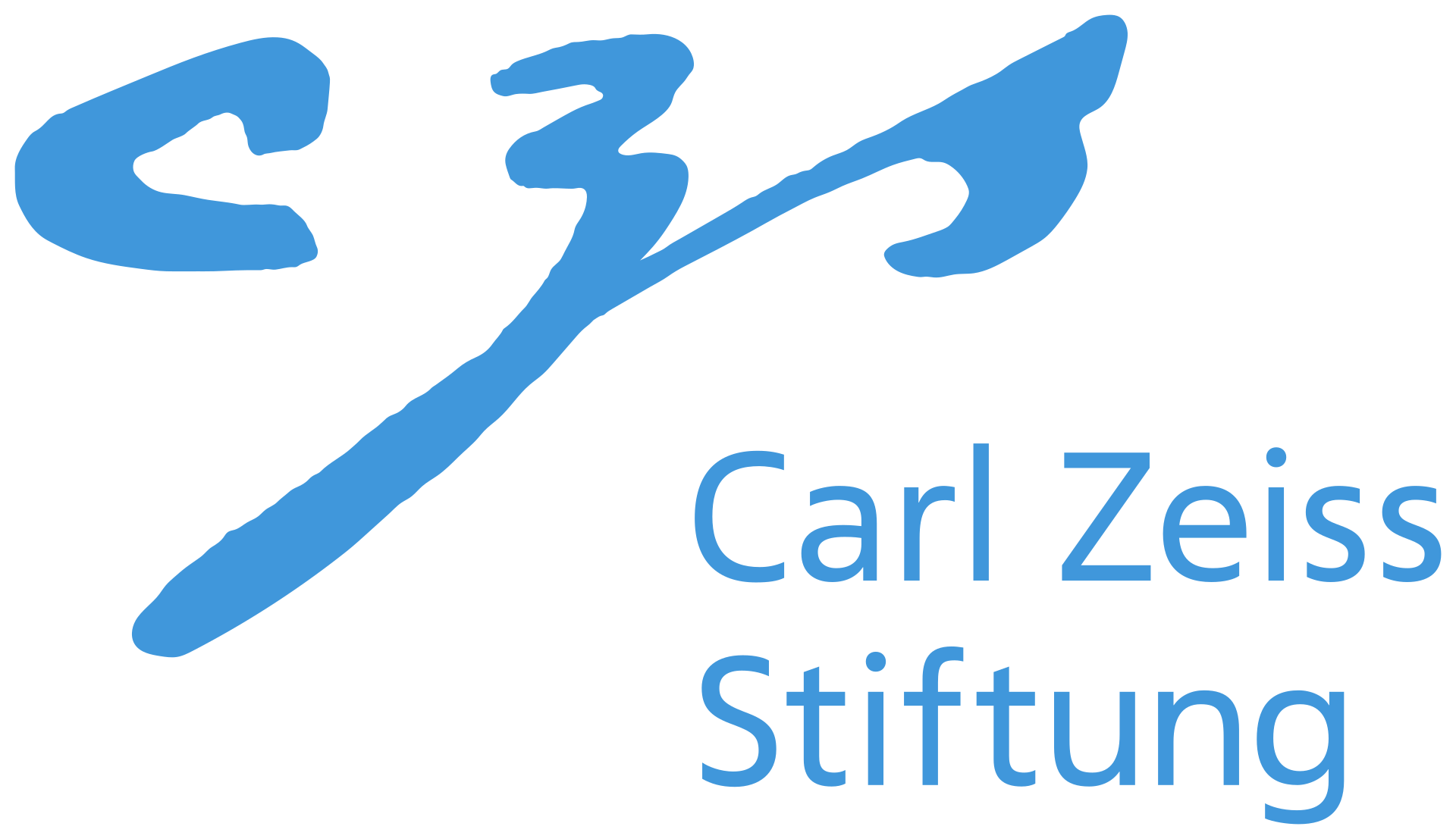
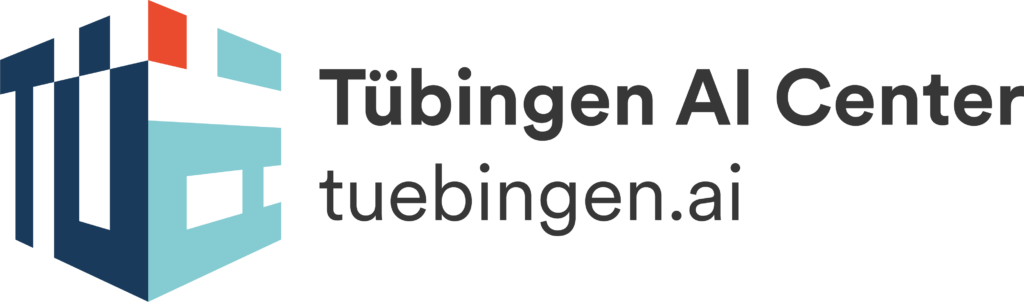
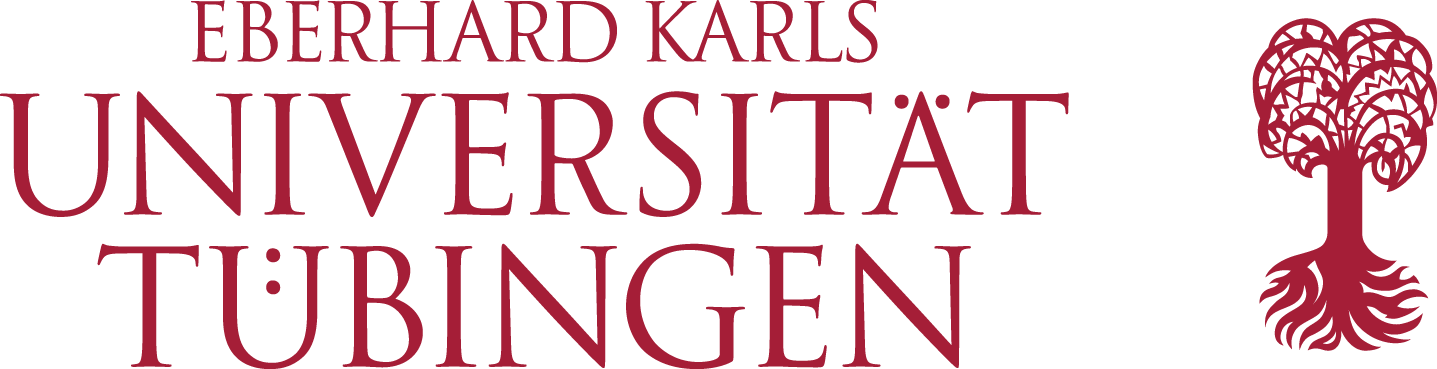
We thank RVH group members for their helpful discussions. We also thank reviewers for their feedback which improves the manuscript. This work is funded by the Deutsche Forschungsgemeinschaft (DFG, German Research Foundation) - 409792180 (Emmy Noether Programme, project: Real Virtual Humans), and German Federal Ministry of Education and Research (BMBF): T¨ubingen AI Center, FKZ: 01IS18039A. Gerard Pons-Moll is a member of the Machine Learning Cluster of Excellence, EXC number 2064/1 – Project number 390727645.The project was made possible by funding from the Carl Zeiss Foundation.