Learning to Transfer Texture from Clothing Images to 3D Humans
Aymen Mir1, Thiemo Alldieck1,2, Gerard Pons-Moll11Max Planck Institute for Informatics, Saarland Informatics Campus, Germany
2Computer Graphics Lab, TU Braunschweig, Germany 
CVPR 2020, Virtual
Paper, Supplementary, Code, Arxiv
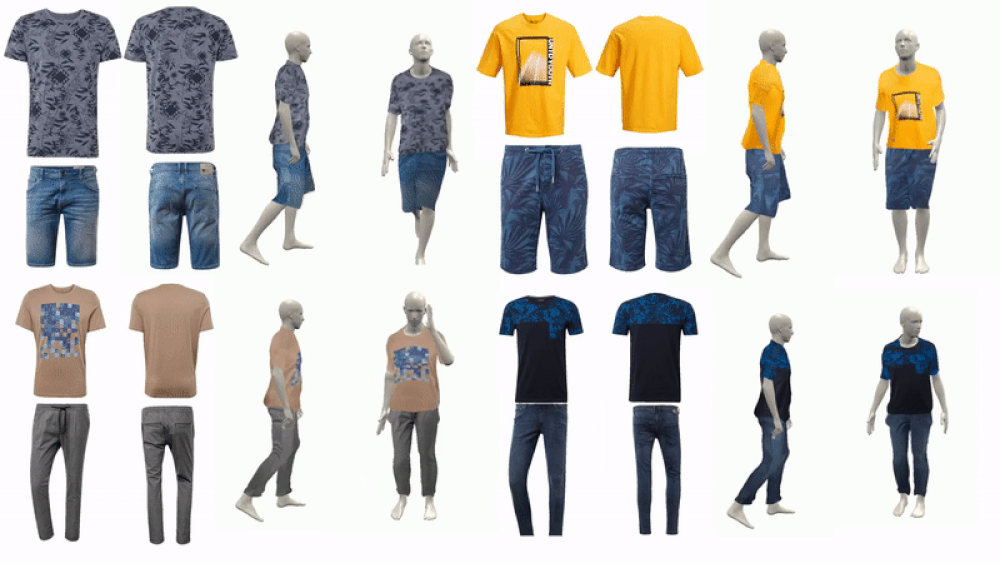
Abstract
In this paper, we present a simple yet effective method to automatically transfer textures of clothing images (front and back) to 3D garments worn on top SMPL, in real time. We first automatically compute training pairs of images with aligned 3D garments using a custom non-rigid 3D to 2D registration method, which is accurate but slow. Using these pairs, we learn a mapping from pixels to the 3D garment surface. Our idea is to learn dense correspondences from garment image silhouettes to a 2D-UV map of a 3D garment surface using shape information alone, completely ignoring texture, which allows us to generalize to the wide range of web images. Several experiments demonstrate that our model is more accurate than widely used baselines such as thin-plate-spline warping and image-to-image translation networks while being orders of magnitude faster. Our model opens the door for applications such as virtual try-on, and allows for generation of 3D humans with varied textures which is necessary for learning.
Code
Citation
@inproceedings{mir20pix2surf, title = {Learning to Transfer Texture from Clothing Images to 3D Humans}, author = {Mir, Aymen and Alldieck, Thiemo and Pons-Moll, Gerard}, booktitle = {{IEEE} Conference on Computer Vision and Pattern Recognition (CVPR)}, month = {June}, organization = {{IEEE}}, year = {2020}, }