Stereo Radiance Fields (SRF): Learning View Synthesis for Sparse Views of Novel Scenes
Julian Chibane1,2, Aayush Bansal3, Verica Lazova1,2, Gerard Pons-Moll1,21University of Tübingen, Germany
2Max Planck Institute for Informatics, Saarland Informatics Campus, Germany
3Carnegie Mellon University, USA
CVPR 2021 Virtual
Overview Video
Abstract & Method
In this work, we introduce Stereo Radiance Fields (SRF), a neural view synthesis approach that is trained end-to-end, generalizes to new scenes in a single forward pass, and requires only sparse views at test time. (b)


SRF intuition: Building on intuition from stereo reconstruction systems, SRF achieves this by composing information of image pairs. 3D points on an opaque, non-occluded surface will project to similar-looking regions when viewed from different perspectives (blue). A point in free space, will not (red).

Citation
@inproceedings{SRF, title = {Stereo Radiance Fields (SRF): Learning View Synthesis from Sparse Views of Novel Scenes }, author = {Chibane, Julian and Bansal, Aayush and Lazova, Verica and Pons-Moll, Gerard}, booktitle = {{IEEE} Conference on Computer Vision and Pattern Recognition (CVPR)}, month = {jun}, organization = {{IEEE}}, year = {2021}, }
Acknowledgments
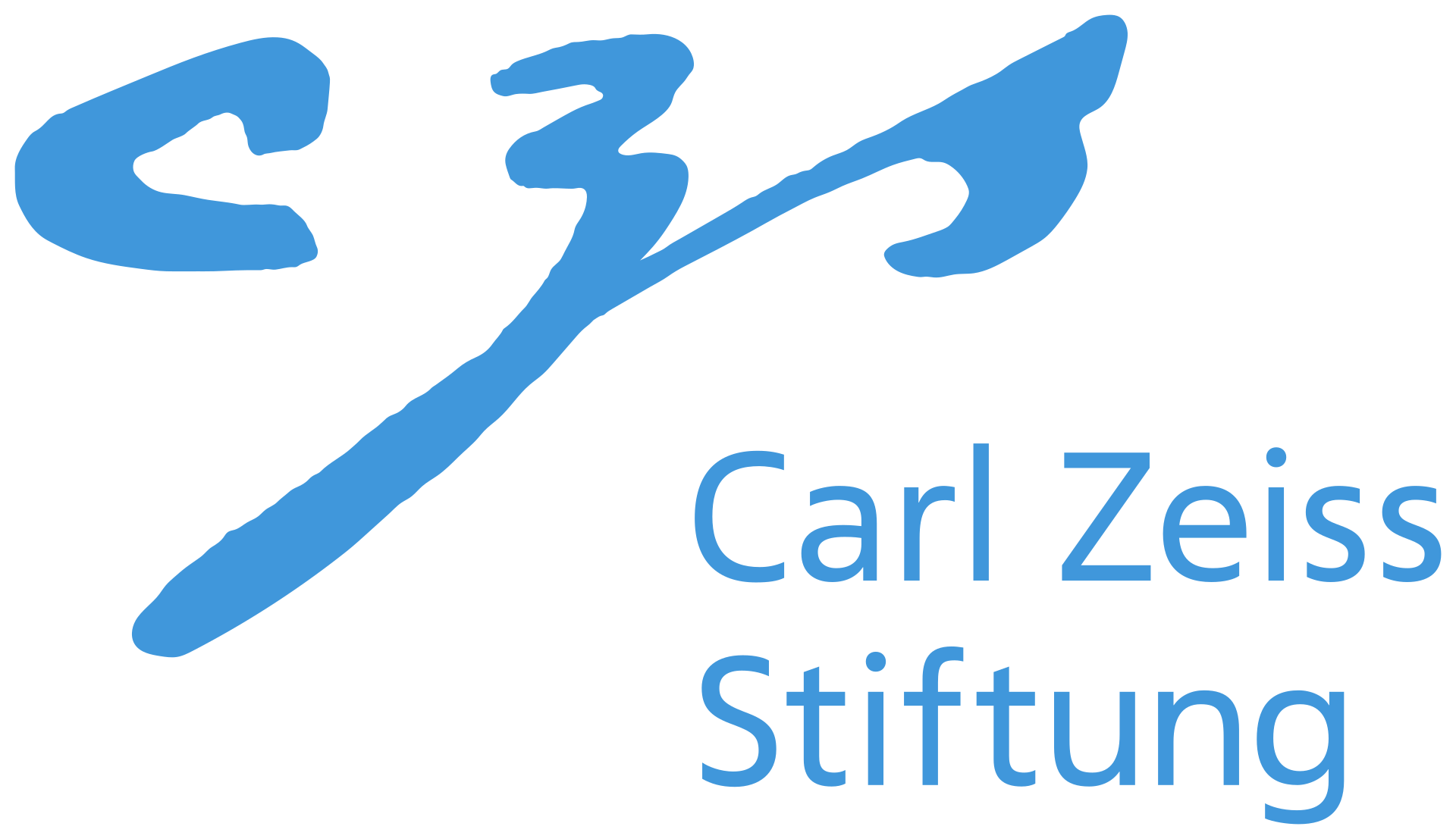
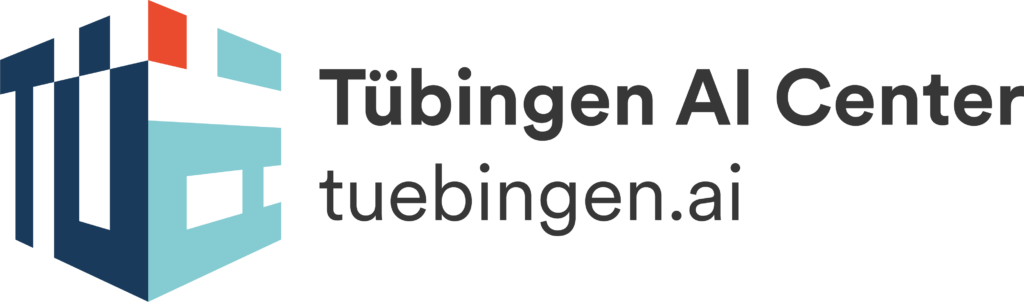
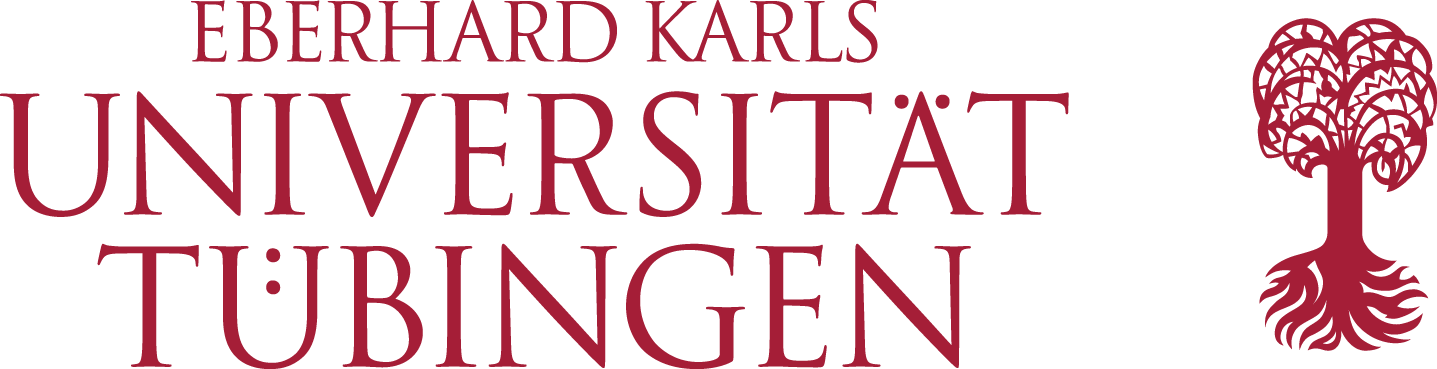
We thank the RVH group for their feedback. This work is funded by the Deutsche Forschungsgemeinschaft (DFG, German Research Foundation) - 409792180 (Emmy Noether Programme, project: Real Virtual Humans). The project was made possible by funding from the Carl Zeiss Foundation.