Generating Continual Human Motion in Diverse 3D Scenes
Aymen Mir1,2, Xavier Puig3, Angjoo Kanazawa4, Gerard Pons-Moll1,21Tubingen AI Center, University of Tubingen, Germany
2Max Planck Institute for Informatics, Saarland Informatics Campus, Germany
3Meta AI Research 
4University of California, Berkeley 
Code, Arxiv
Abstract
We introduce a method to synthesize animator guided human motion across 3D scenes. Given a set of sparse (3 or 4) joint locations (such as the location of a person's hand and two feet) and a seed motion sequence in a 3D scene, our method generates a plausible motion sequence starting from the seed motion while satisfying the constraints imposed by the provided keypoints. We decompose the continual motion synthesis problem into walking along paths and transitioning in and out of the actions specified by the keypoints, which enables long generation of motions that satisfy scene constraints without explicitly incorporating scene information. Our method is trained only using scene agnostic mocap data. As a result, our approach is deployable across 3D scenes with various geometries. For achieving plausible continual motion synthesis without drift, our key contribution is to generate motion in a goal-centric canonical coordinate frame where the next immediate target is situated at the origin. Our model can generate long sequences of diverse actions such as grabbing, sitting and leaning chained together in arbitrary order, demonstrated on scenes of varying geometry: HPS, Replica, Matterport, ScanNet and scenes represented using NeRFs. Several experiments demonstrate that our method outperforms existing methods that navigate paths in 3D scenes.
Overview.
Our method takes a set of keypoints as input
Our method synthesize motion in diverse 3D scenes.
Diverse Actions.
Code
Citation
@inproceedings{mir23origin, title = {Generating Continual Human Motion in Diverse 3D Scenes}, author = {Mir, Aymen and Puig, Xavier and Kanazawa, Angjoo and Pons-Moll, Gerard}, booktitle = {International Conference on 3D Vision (3DV)}, month = {March}, year = {2024}, }
Acknowledgments
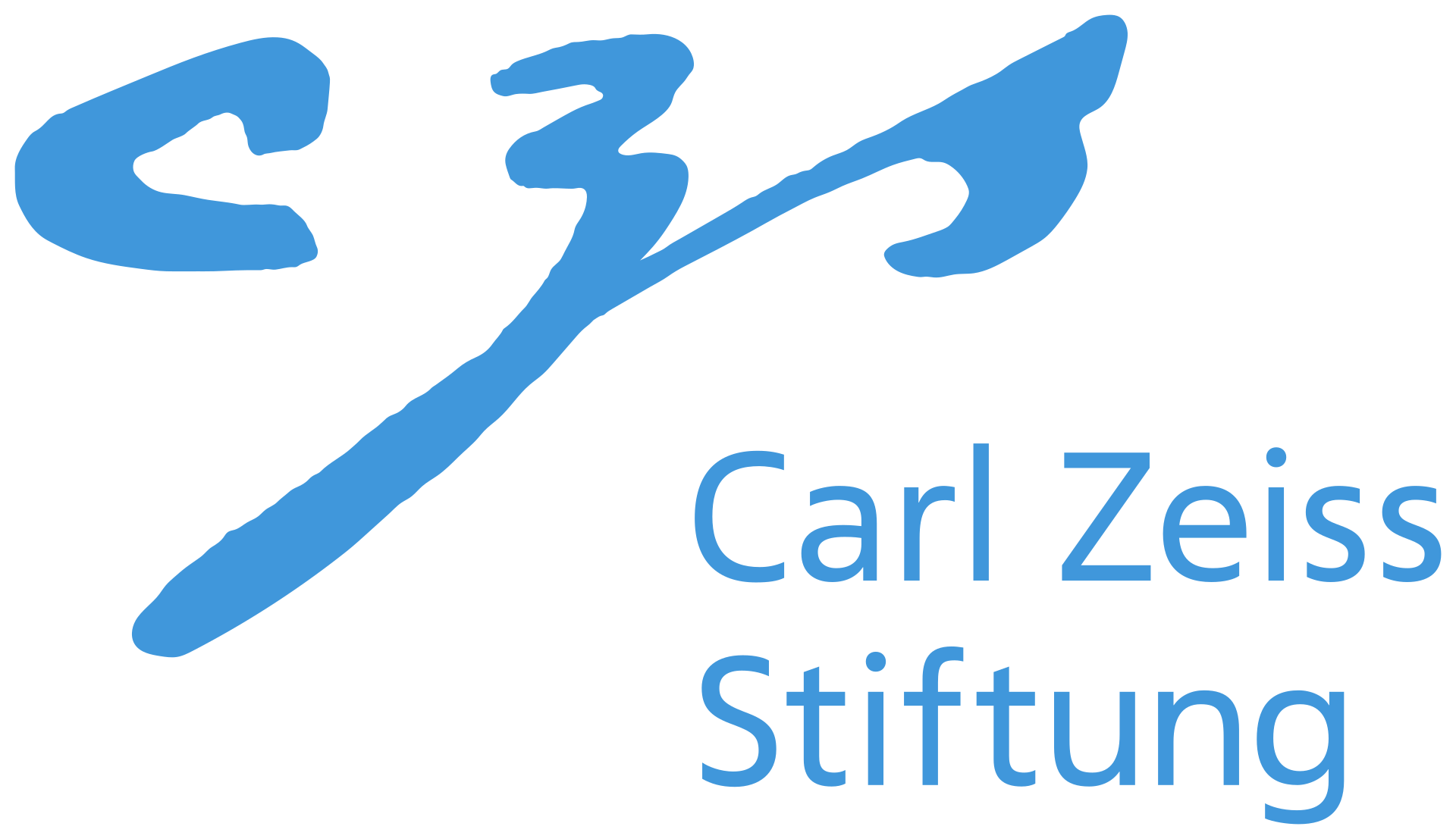
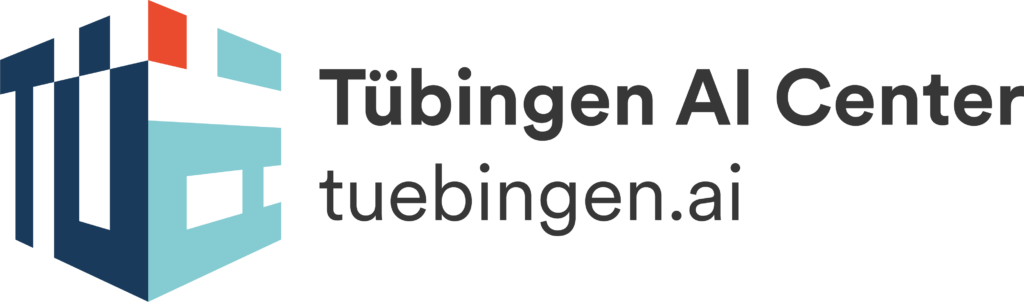
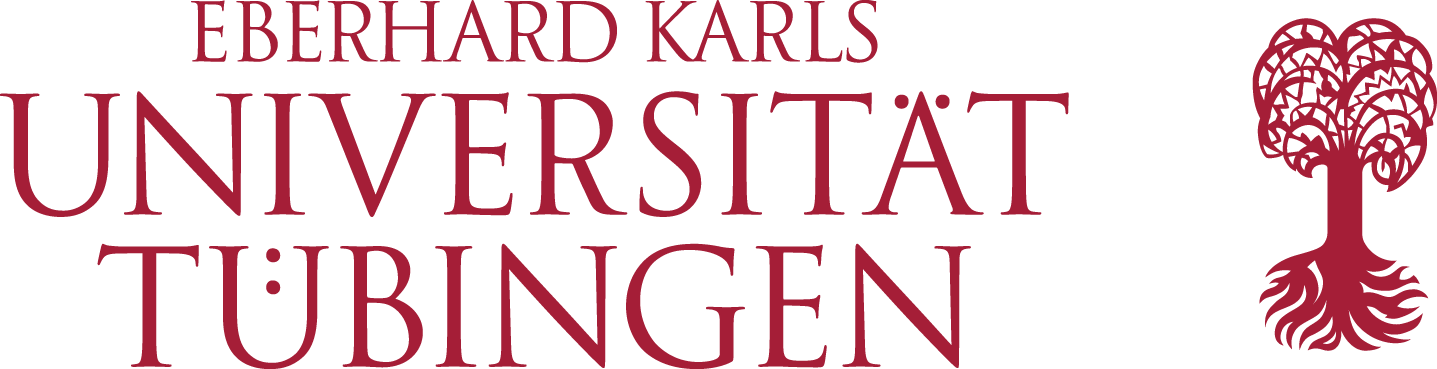
This work is funded by the Deutsche Forschungsgemeinschaft (DFG, German Research Foundation) - 409792180 (Emmy Noether Programme, project: Real Virtual Humans) and German Federal Ministry of Education and Research (BMBF): T¨ubingen AI Center, FKZ: 01IS18039A. Gerard Pons-Moll is a member of the Machine Learning Cluster of Excellence, EXC number 2064/1 – Project number 390727645. The project was made possible by funding from the Carl Zeiss Foundation.