GEARS: Local Geometry-aware Hand-object Interaction Synthesis
Keyang Zhou 1, 2 , Bharat Lal Bhatnagar 1, 2 , Jan Eric Lenssen 2 , Gerard Pons-Moll 1, 21University of Tübingen, Germany
2Max Planck Institute for Informatics, Saarland Informatics Campus, Germany
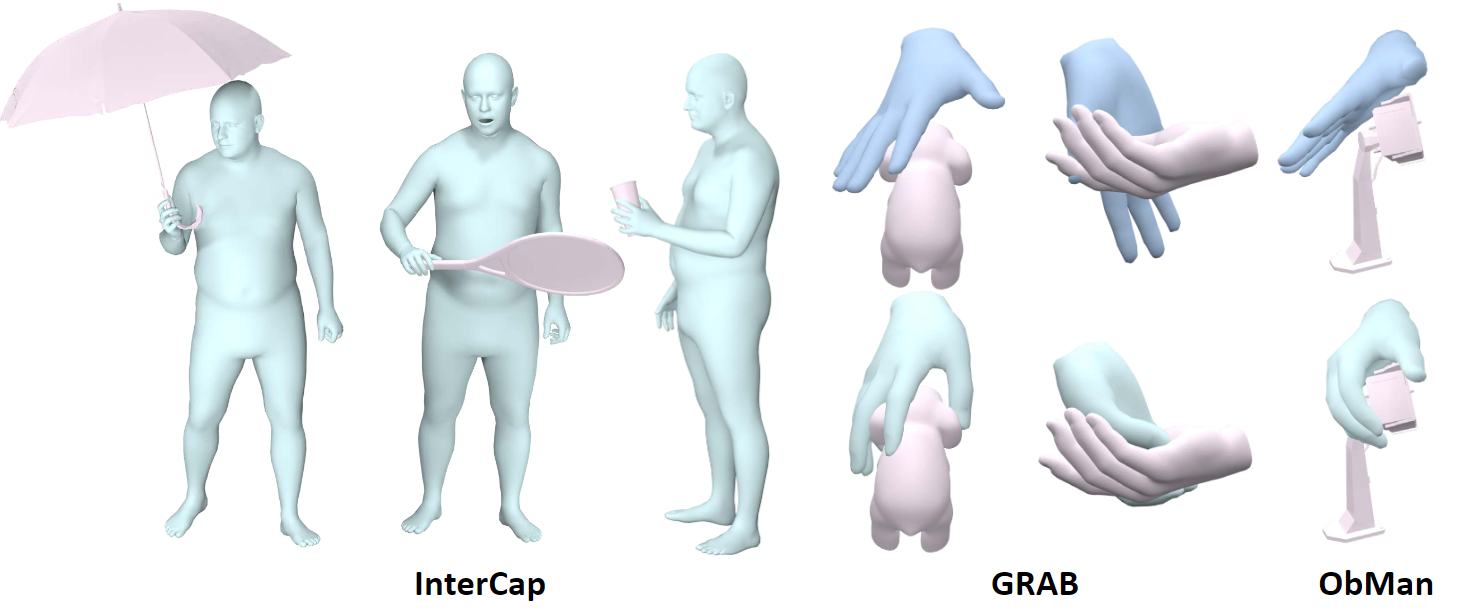
We propose GEARS, a method to synthesize sequence of hand poses during interaction with an object. GEARS takes hand and object trajectory as input. It generates realistic hand poses that are well-adapted to object surface, irrespective of object category and size. In the figure above, hands colored in blue are inputs while hands colored in cyan are our predictions.
Local Geometry Sensor
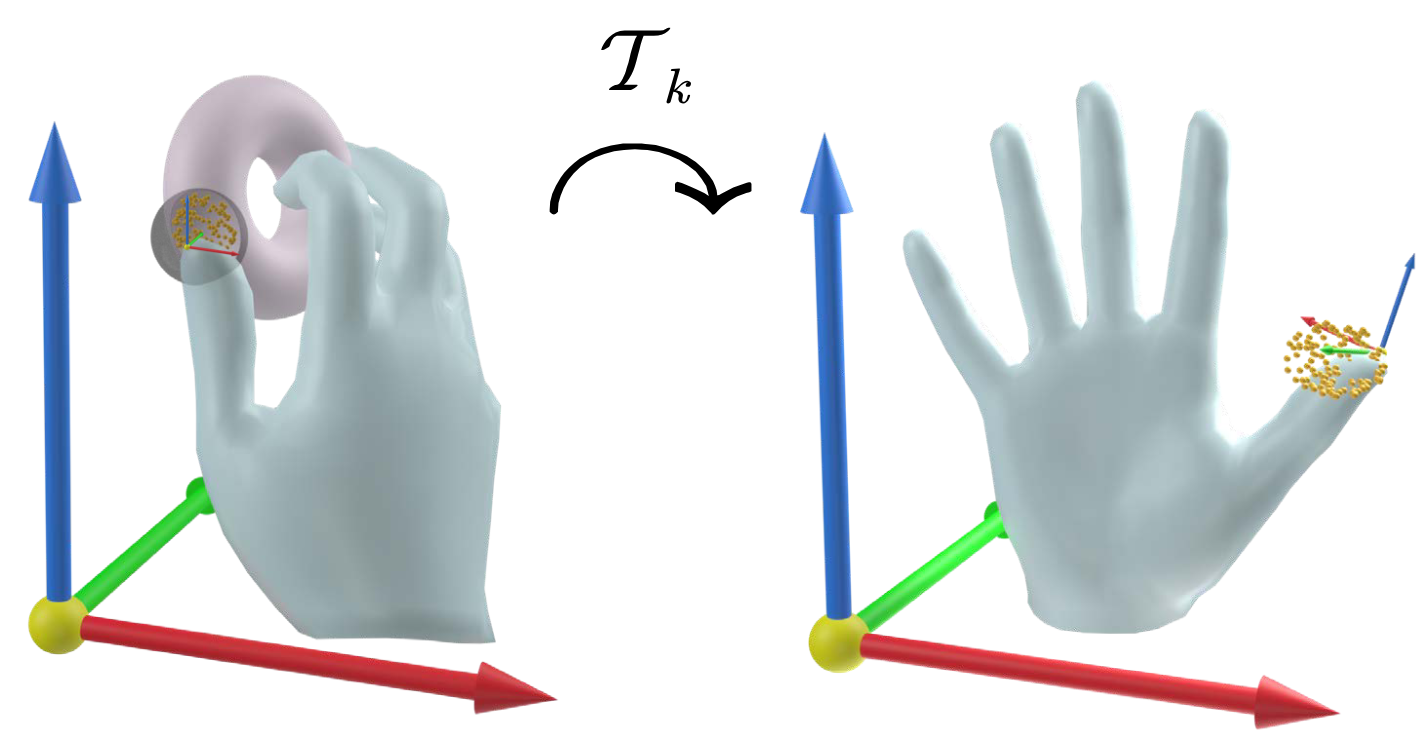
Given the joints positions and the object mesh, we sample points on the object surface within a specified radius centered at each joint. The object points are represented in a joint-local frame. To promote better generalization, we transform the sampled object points from global frame to the canonical frame defined by the MANO template hand.
Spatio-temporal Transformer
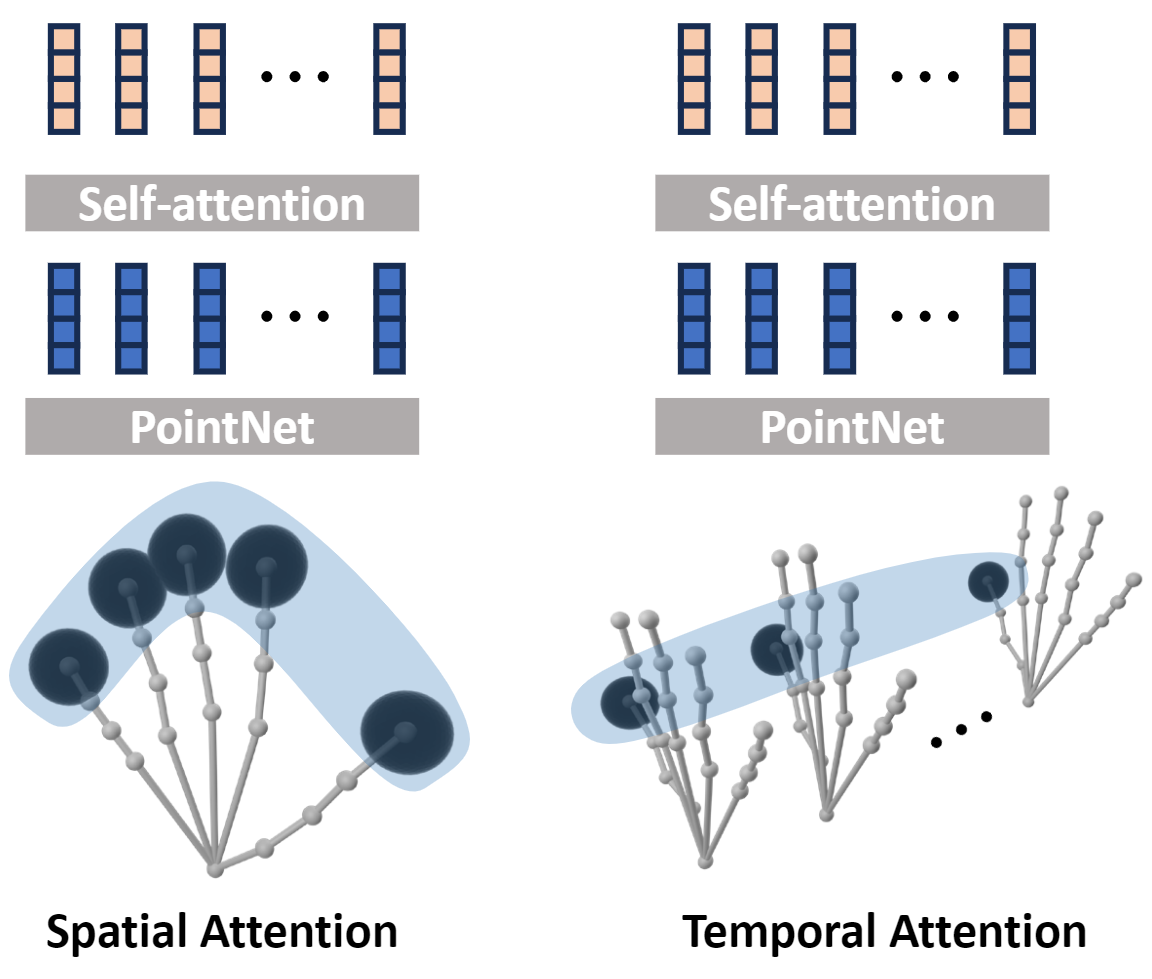
For spatial attention, every joint attends to every other joint of the same hand. This module takes the hands in different frames as static identities, and focuses on learning the correlations between different fingers. While for temporal attention, a joint in one frame attends to the same joint in every other frame. This module models the trajectory of each individual joint, ensuring that all joints move in a temporally smooth and consistent manner.
Qualitative Results
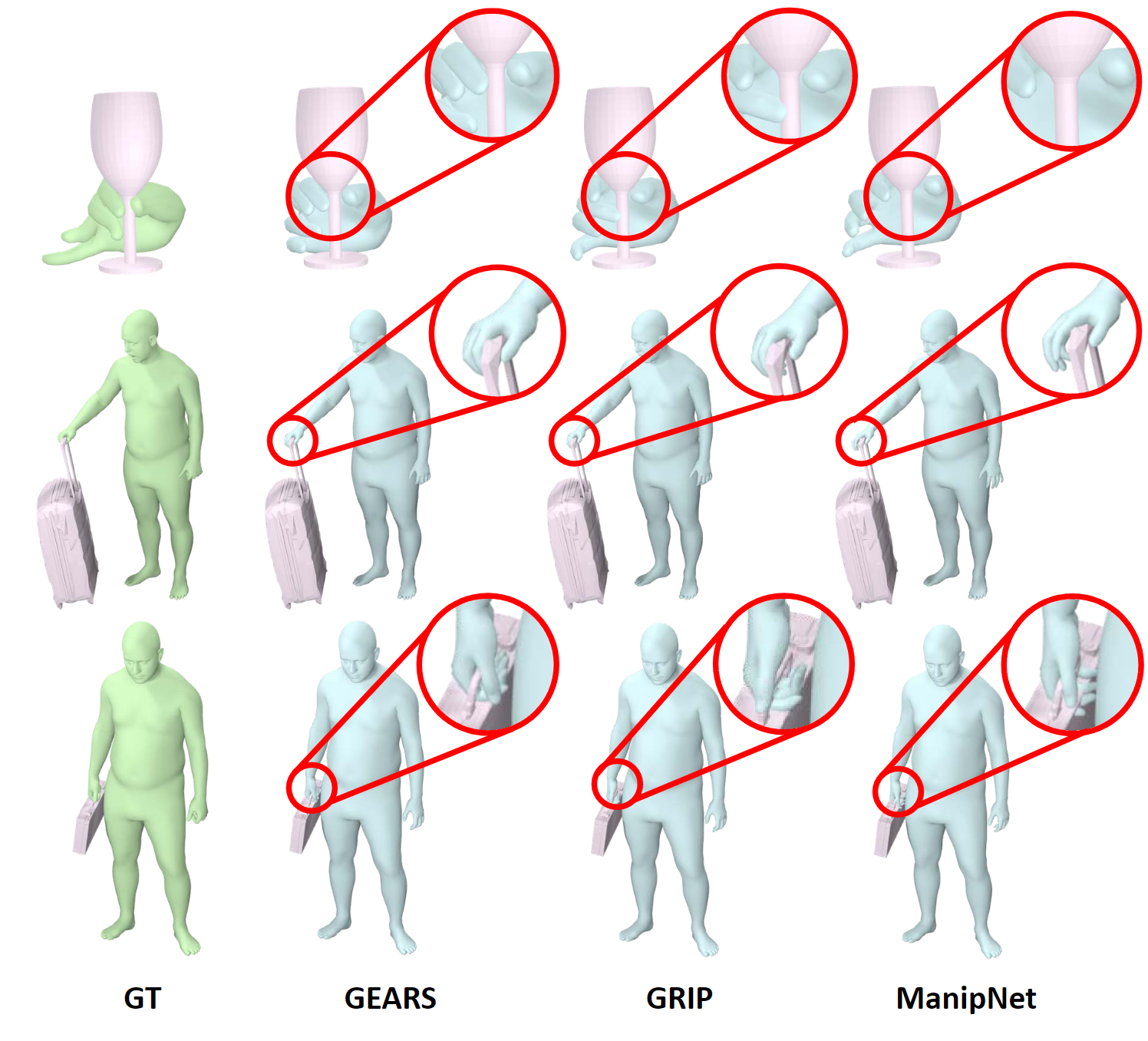
Citation
@inproceedings{zhou2024gears, title = {GEARS: Local Geometry-aware Hand-object Interaction Synthesis}, author = {Zhou, Keyang and Bhatnagar, Bharat Lal and Lenssen, Jan Eric and Pons-Moll, Gerard}, booktitle = {IEEE Conference on Computer Vision and Pattern Recognition (CVPR)}, month = {June}, year = {2024}, }
Acknowledgments
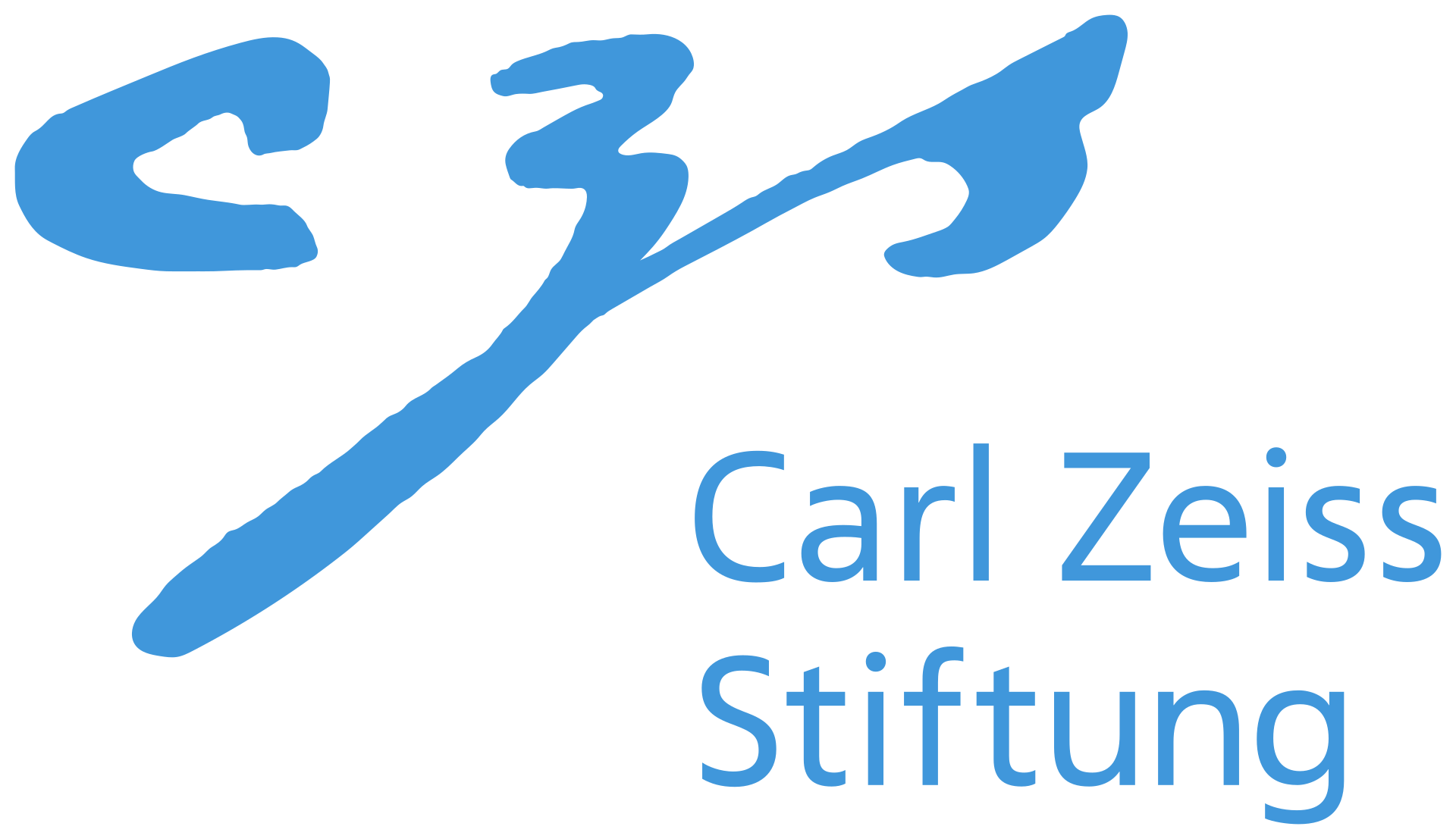
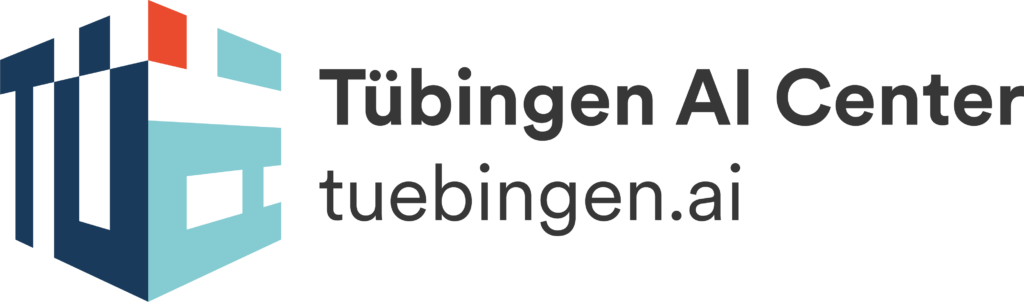
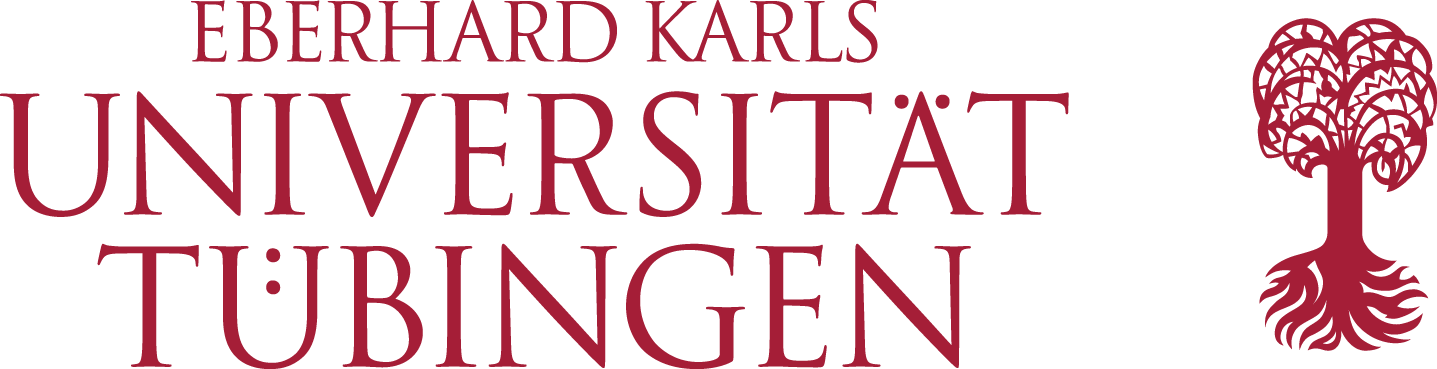
This work is supported by the German Federal Ministry of Education and Research (BMBF): T¨ubingen AI Center, FKZ: 01IS18039A. This work is funded by the Deutsche Forschungsgemeinschaft (DFG, German Research Foundation) - 409792180 (Emmy Noether Programme, project: Real Virtual Humans). Gerard Pons-Moll is a member of the Machine Learning Cluster of Excellence, EXC number 2064/1 – Project number 390727645. The project was made possible by funding from the Carl Zeiss Foundation.