Box2Mask: Weakly Supervised 3D Semantic Instance Segmentation Using Bounding Boxes
Julian Chibane1,2, Francis Engelmann3, Tuan Anh Tran2, Gerard Pons-Moll1,21University of Tübingen, Germany
2Max Planck Institute for Informatics, Saarland Informatics Campus, Germany
3ETH Zurich AI Center, Switzerland
European Conference on Computer Vision (ECCV), 2022 - Oral
Are 3D bounding box annotations suitable to train dense 3D semantic instance segmentation?
Yes!

Key Finding

Box2Mask - Method Overview
Results
Box2Mask trained via bounding boxes, obtains result quality of fully supervised SOTA methods (96.9% of full supervision in mAP@50, 100.0% in mAP@25) on the ScanNet benchmark, and largly outperforms prior weakly supervised methods (+ 15.2 mAP@50). See the paper for details.Interactive Results
Object semantics:
Cabinet
Bed
Chair
Sofa
Table
Door
Window
Bookshelf
Picture
Counter
Desk
Curtain
Refrigerator
Bathtub
Shower curtain
Toilet
Sink
Other furniture
Object instances: Different colors (chosen at random) represent different instances.
Video
Citation
@inproceedings{chibane2021box2mask, title = {Box2Mask: Weakly Supervised 3D Semantic Instance Segmentation Using Bounding Boxes}, author = {Chibane, Julian and Engelmann, Francis and Tran, Tuan Anh and Pons-Moll, Gerard}, booktitle = {European Conference on Computer Vision ({ECCV})}, month = {October}, organization = {{Springer}}, year = {2022}, }
Acknowledgments
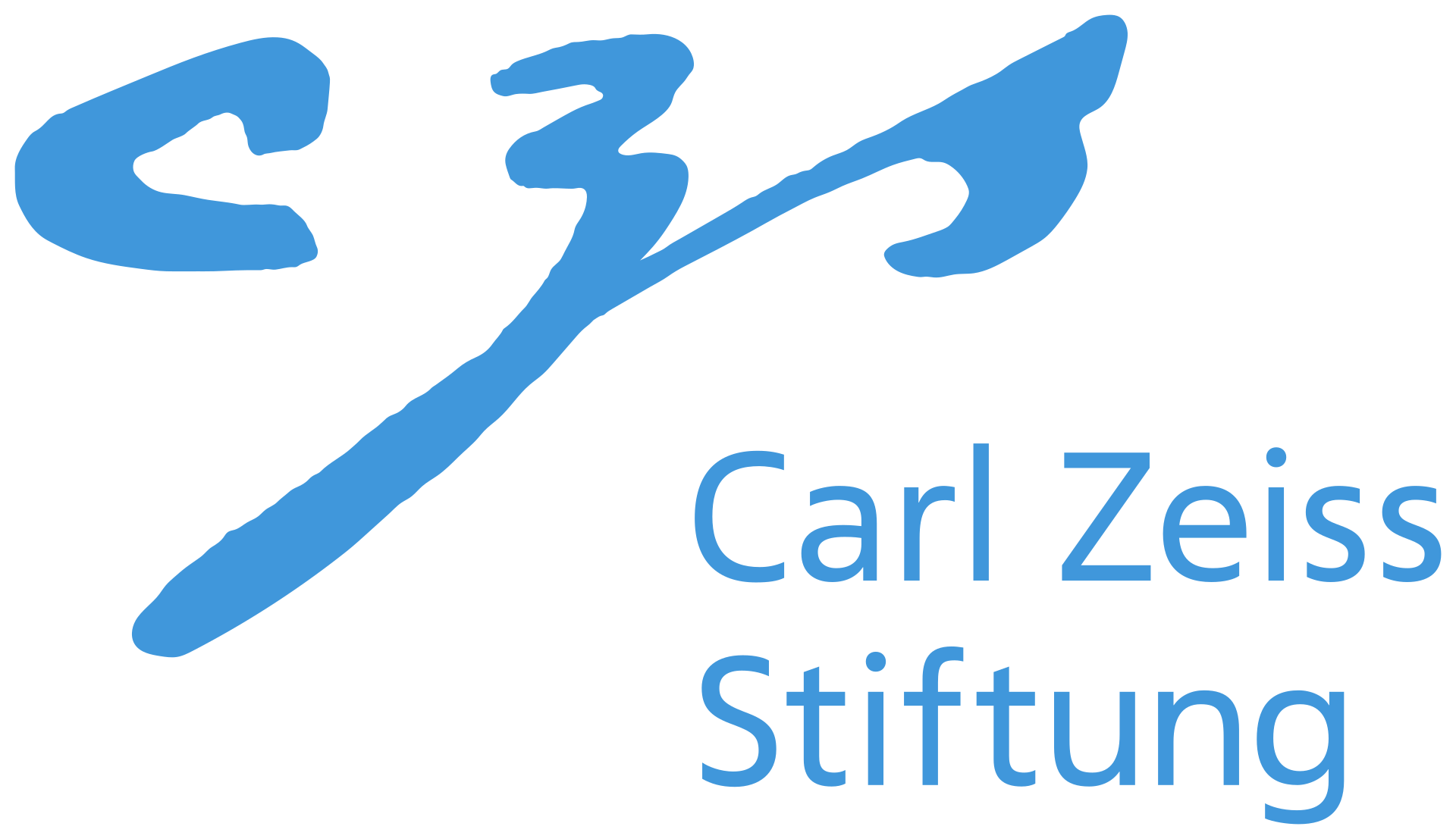
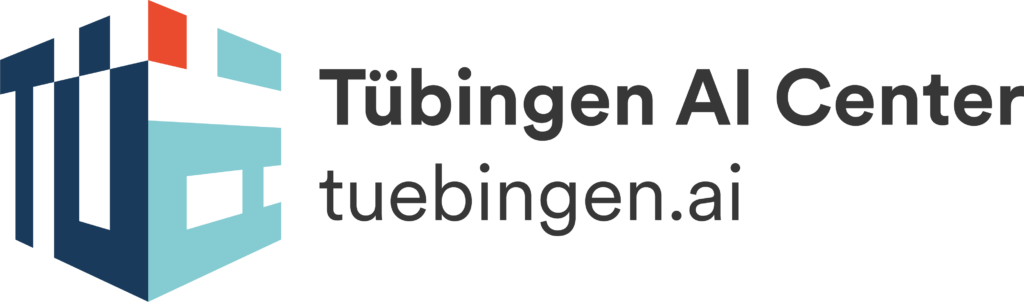
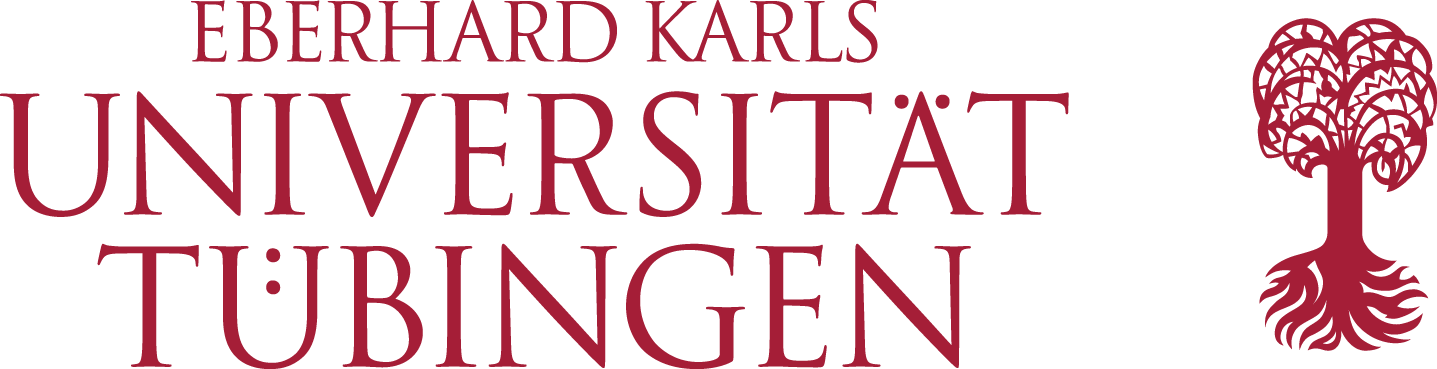
We thank Alexey Nekrasov and Jonas Schult for helpful discussions and feedback. This work is funded by the Deutsche Forschungsgemeinschaft (DFG, German Research Foundation) - 409792180 (Emmy Noether Programme, project: Real Virtual Humans). Gerard Pons-Moll is a member of the Machine Learning Cluster of Excellence, EXC number 2064/1 – Project number 390727645. Julian Chibane is a fellow of the Meta Research PhD Fellowship Program - area: AR/VR Human Understanding. Francis Engelmann is a post-doctoral research fellow at the ETH AI Center. The project was made possible by funding from the Carl Zeiss Foundation.