TOCH: Spatio-Temporal Object-to-Hand Correspondence for Motion Refinement
Keyang Zhou 1, 2 , Bharat Lal Bhatnagar 1, 2 , Jan Eric Lenssen 2 , Gerard Pons-Moll 1, 21University of Tübingen, Germany
2Max Planck Institute for Informatics, Saarland Informatics Campus, Germany

We present TOCH, a method for refining incorrect 3D hand-object interaction sequences using a data prior. Existing hand trackers, especially those that rely on very few cameras, often produce visually unrealistic results with hand-object intersection or missing contacts. Although correcting such errors requires reasoning about temporal aspects of interaction, most previous work focus on static grasps and contacts. The core of our method are TOCH fields, a novel spatio-temporal representation for modeling correspondences between hands and objects during interaction. The key component is a point-wise object-centric representation which encodes the hand position relative to the object. Leveraging this novel representation, we learn a latent manifold of plausible TOCH fields with a temporal denoising auto-encoder. Experiments demonstrate that TOCH outperforms state-of-the-art (SOTA) 3D hand-object interaction models, which are limited to static grasps and contacts. More importantly, our method produces smooth interactions even before and after contact. Using a single trained TOCH model, we quantitatively and qualitatively demonstrate its usefulness for 1) correcting erroneous reconstruction results from off-the-shelf RGB/RGB-D hand-object reconstruction methods, 2) de-noising, and 3) grasp transfer across objects.
Motion Refinement
Grasp Transfer
Citation
@inproceedings{zhou2022toch, title = {TOCH: Spatio-Temporal Object-to-Hand Correspondence for Motion Refinement}, author = {Zhou, Keyang and Bhatnagar, Bharat Lal and Lenssen, Jan Eric and Pons-Moll, Gerard}, booktitle = {European Conference on Computer Vision ({ECCV})}, month = {October}, organization = {{Springer}}, year = {2022}, }
Acknowledgments
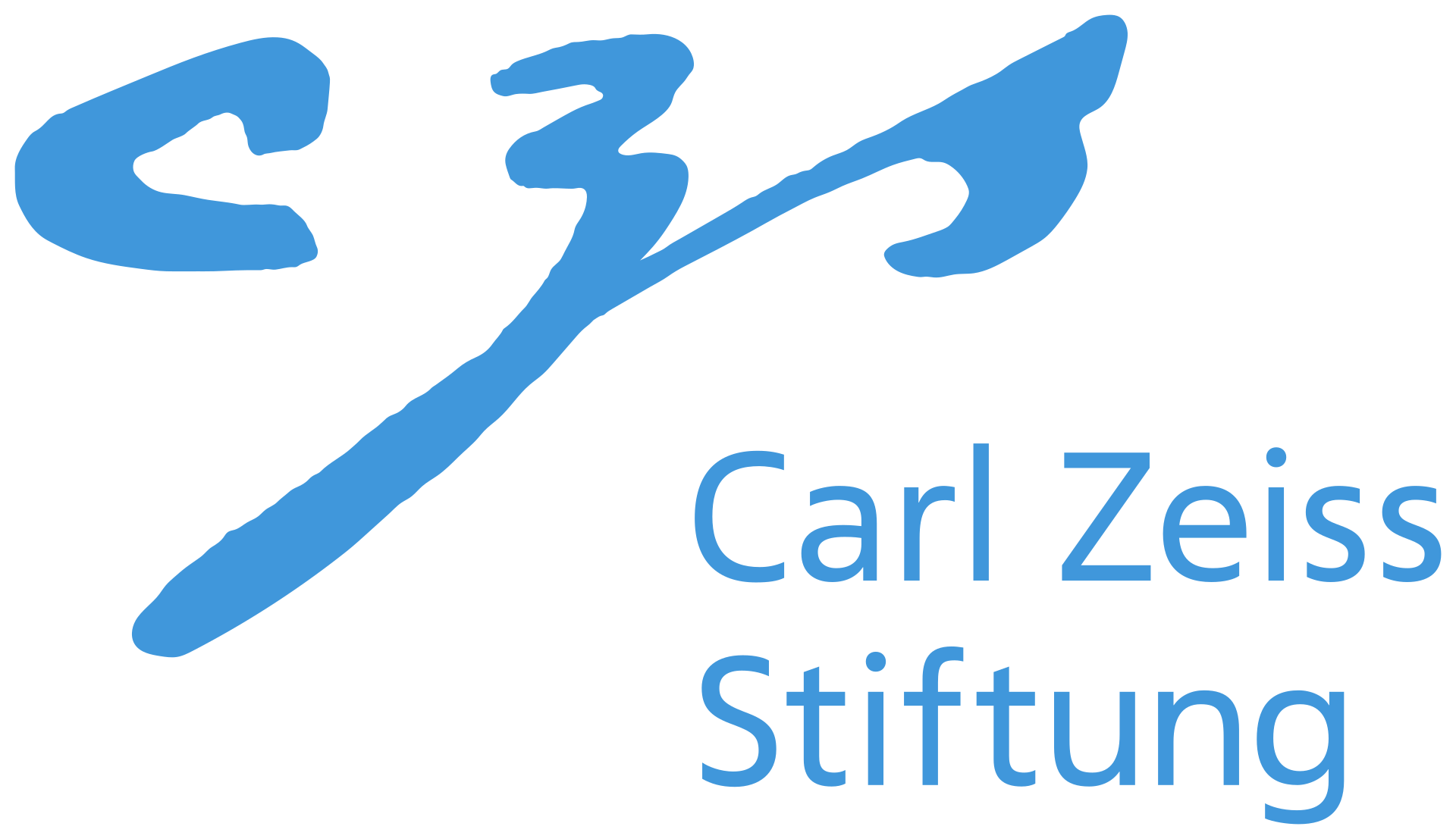
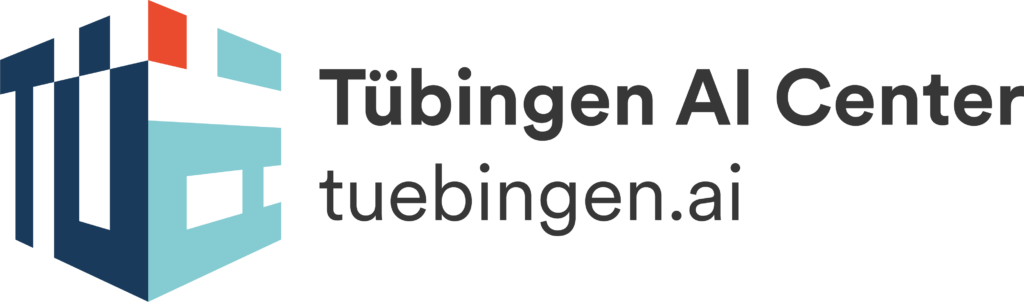
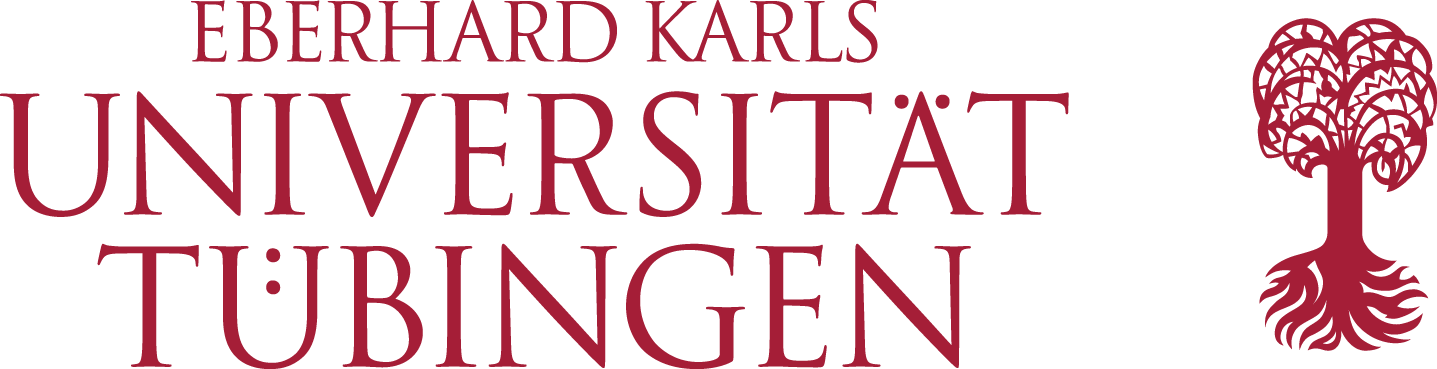
This work is supported by the German Federal Ministry of Education and Research (BMBF): T¨ubingen AI Center, FKZ: 01IS18039A. This work is funded by the Deutsche Forschungsgemeinschaft (DFG, German Research Foundation) - 409792180 (Emmy Noether Programme, project: Real Virtual Humans). Gerard Pons-Moll is a member of the Machine Learning Cluster of Excellence, EXC number 2064/1 – Project number 390727645. The project was made possible by funding from the Carl Zeiss Foundation.